Active Learning. Burr Settles
Чтение книги онлайн.
Читать онлайн книгу Active Learning - Burr Settles страница 6
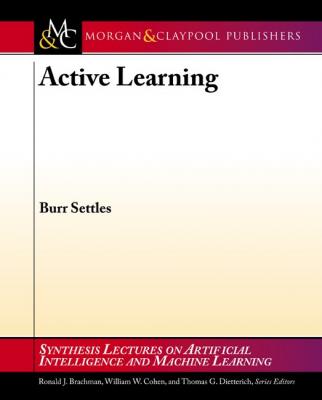
Stream-Based Selective Sampling. An alternative to synthesizing queries is selective sampling (Atlas et al., 1990; Cohn et al., 1994). The key assumption is that obtaining an unlabeled instance is free (or inexpensive), so it can first be sampled from the actual distribution, and then the learner can decide whether or not to request its label. The approach is illustrated in Figure 1.5(a). This is sometimes called stream-based active learning, as each unlabeled instance is typically drawn one at a time from the input source, and the learner must decide whether to query or discard it. If the input distribution is uniform, selective sampling may not offer any particular advantages over query synthesis. However, if the distribution is non-uniform and (more importantly) unknown, we are guaranteed that queries will still be sensible, since they come from a real underlying distribution.
Figure 1.5: (a) In selective sampling, the learner decides whether to query or discard items from a stream of input instances. (b) Pool-based active learning queries the most informative instance from a large pool of available unlabeled data U.
The decision whether to query or discard an instance can be framed several ways. One approach is to define a measure of utility or information content (several such measures are discussed in Chapters 2–5) and make a biased random decision, such that instances with higher utility are more likely to be queried (c.f., Dagan and Engelson, 1995). Another approach is to compute an explicit region of uncertainty (Cohn et al., 1994), i.e., the part of the instance space that is still ambiguous to the learner, and only query instances that fall within it. A naive way of doing this is to set a minimum threshold on a utility measure which defines the region. Instances whose evaluation is above this threshold are then queried. Another somewhat more principled approach is to define the region that is still unknown to the overall model class, i.e., to the set of hypotheses consistent with the current labeled training set called the version space (Mitchell, 1982). In other words, if any two models of the same model class (but different parameter settings) agree on all the labeled data, but disagree on an unlabeled instance sampled from the data source, then that instance lies within the region of uncertainty. Calculating this region completely and explicitly is computationally expensive, however, and it must be maintained after each new query. As a result, approximations are used in practice (more details in Chapter 3).
The stream-based scenario has been studied in several real-world tasks, including part-of-speech tagging (Dagan and Engelson, 1995), sensor scheduling (Krishnamurthy,2002), and learning ranking functions for information retrieval (Yu, 2005). Fujii et al. (1998) employed selective sampling for active learning in word sense disambiguation, e.g., determining if the word “bank” means land alongside a river or a financial institution in a given context (except they study Japanese words in their work). The approach not only reduces annotation effort, but also limits the size of the database used in nearest-neighbor learning, which in turn expedites the classification algorithm.
It is worth noting that some authors (e.g., Moskovitch et al., 2007; Thompson et al., 1999) use the term “selective sampling” to refer to the pool-based scenario described next. Under this interpretation, the term merely signifies that queries are made with a selected subset of instances sampled from a real data distribution. However, in most of the literature selective sampling refers to the stream-based scenario described here.
Pool-Based Sampling. For many real-world learning problems, large collections of unlabeled data can be gathered at once. This motivates pool-based sampling (Lewis and Gale, 1994), which assumes that there is a small set of labeled data L and a large pool of unlabeled data U available. The approach is illustrated in Figure 1.5(b). Queries are selected from the pool, which is usually assumed to be closed (i.e., static or non-changing), although this is not strictly necessary. Queries are typically chosen in a greedy fashion, according to a utility measure used to evaluate all instances in the pool (or, perhaps if U is very large, a subsample thereof). The binary search algorithm for the alien fruits example in Section 1.1 is a pool-based active learning algorithm.
The pool-based scenario has been studied for many real-world problem domains in machine learning, such as text classification (Hoi et al., 2006a; Lewis and Gale, 1994; McCallum and Nigam, 1998; Tong and Koller, 2000), information extraction (Settles and Craven, 2008; Thompson et al., 1999), image classification and retrieval (Tong and Chang, 2001; Zhang and Chen, 2002), video classification and retrieval (Hauptmann et al., 2006; Yan et al., 2003), speech recognition (Tür et al., 2005), and cancer diagnosis (Liu, 2004), to name only a few. In fact, pool-based sampling appears to be the most popular scenario for applied research in active learning, whereas query synthesis and stream-based selective sampling are more common in the theoretical literature.
The main difference between stream-based and pool-based active learning is that the former obtains one instance at a time, sequentially from some streaming data source (or by scanning through the data) and makes each query decision individually. Pool-based active learning, on the other hand, evaluates and ranks the entire collection of unlabeled data before selecting the best query. While the pool-based scenario appears to be much more common among application papers, one can imagine settings where the stream-based approach is more appropriate. For example, when memory or processing power is limited, as with mobile and embedded devices, or when the data set is too large to load into memory and must be scanned sequentially from disk. Unless otherwise noted, however, we will assume a pool-based scenario for our discussion of the algorithms discussed in the remainder of this book.
1More detail on PAC learning and active learning will be discussed in Chapter 6.
2Relaxations of these and other assumptions are discussed in Chapter 7.
CHAPTER 2
Uncertainty Sampling
“Information is the resolution of uncertainty.”
— Claude Shannon
2.1 PUSHING THE BOUNDARIES
Let us revisit the alien fruits problem from Section 1.1, and use it as a running example. Recall that we want to efficiently test fruits for ⊕ safe
vs. ⊖ noxious
to eat. One solution is to lay out all the fruits in a line from most round to most irregular, and use a binary search algorithm to actively select which fruits to test. This approach is fine for our simple thought experiment, but we want a more general search algorithm for arbitrary problems with many input dimensions, potentially many choices for output (e.g., multiple class labels