Communication Networks and Service Management in the Era of Artificial Intelligence and Machine Learning. Группа авторов
Чтение книги онлайн.
Читать онлайн книгу Communication Networks and Service Management in the Era of Artificial Intelligence and Machine Learning - Группа авторов страница 3
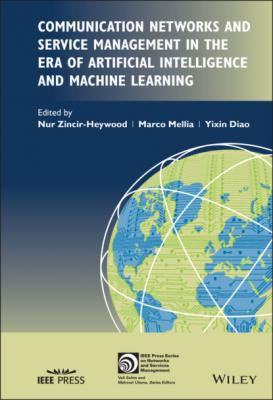
3 Chapter 3Figure 3.1 Technologies for virtualizing network functions with examples.Figure 3.2 Link virtualization technologies with examples.Figure 3.3 Examples of network slices.
4 Chapter 4Figure 4.1 Slicing and prioritization of 5G network traffic. An eHealth netw...Figure 4.2 O‐RAN high‐level architecture.Figure 4.3 vrAIn's control loop.Figure 4.4 5G‐PPP network architecture.
5 Chapter 5Figure 5.1 Overview of AI/ML use cases in a service‐aware 5G integrated netw...Figure 5.2 Possible framework for ML integration in 5G.Figure 5.3 Overview of the prediction process.Figure 5.4 Mean per class accuracy on the testing set when using different a...Figure 5.5 Components of the QoE management framework.
6 Chapter 6Figure 6.1 Brief overview of ML techniques.
7 Chapter 7Figure 7.1 High‐level view of a fog computing environment [6].Figure 7.2 An example of a service function chaining deployment [14].Figure 7.3 The representation schema of most RL scenarios.Figure 7.4 The fog–cloud infrastructure for the gym‐fog environment evaluati...Figure 7.5 The OpenAi gym environment structure.Figure 7.6 The accumulated reward and the cost difference for the static use...Figure 7.7 The percentage of accepted requests for the static scenario.Figure 7.8 The execution time of each episode run.Figure 7.9 The accumulated reward and the cost difference for the dynamic ca...Figure 7.10 The percentage of accepted requests for the dynamic scenario.
8 Chapter 8Figure 8.1 Comparison between (a) traditional and (b) ML/AI‐based approaches...Figure 8.2 Problem instances are first represented as graph. The graph is th...
9 Chapter 9Figure 9.1 Comparison between Bayesian optimization and random search to rea...Figure 9.2 A taxonomy of anomaly detection techniques generalizing the ones ...Figure 9.3 The performance of ANNs models that is trained with training data...Figure 9.4 Streaming workload in a simulated experiment (a) and average rewa...
10 Chapter 10Figure 10.1 A general reinforcement learning setting using neural network as...Figure 10.2 Current DRL systems are insufficient.Figure 10.3 AuTO overview.Figure 10.4 Multi‐level feedback queuing.Figure 10.5 AuTO: A four‐queue example.Figure 10.6 Comparison of deep stochastic and deep deterministic policies.Figure 10.7 Testbed topology.Figure 10.8 Traffic distributions in evaluation.Figure 10.9 Homogeneous traffic: Average and p99 FCT.Figure 10.10 Spatially heterogeneous traffic: Average and p99 FCT.Figure 10.11 Dynamic scenarios: average FCT.Figure 10.12 Dynamic scenarios: p99 FCT.Figure 10.13 MLFQ thresholds from sRLA vs. optimal thresholds.Figure 10.14 Average FCT using MLFQ thresholds from sRLA vs. optimal thresho...Figure 10.15 p99 FCT using MLFQ thresholds from sRLA vs. optimal thresholds....Figure 10.16 Load balancing using lRLA (PG algorithm): difference in number ...Figure 10.17 CS response latency: Traces from four runs.Figure 10.18 CS response latency: Scaling short flows (
11 Chapter 11Figure 11.1 A taxonomy for anomaly detection problems and techniques.Figure 11.2 Examples of anomaly macro‐categories. (a) Pointwise; (b) Context...Figure 11.3 Example of deep neural network with four hidden layers.Figure 11.4 Basic autoencoder structure.Figure 11.5 Basic generative adversarial network architecture.
12 Chapter 12Figure 12.1 Example of a Kinetic control plane automaton.Figure 12.2 Security chain orchestrator integrated into a SDN infrastructure...Figure 12.3 Different steps of the proposed methodology.Figure 12.4 Inferred Markov chain of the Pokemon Go Android application.Figure 12.5 A toy security chain in pyretic and its encoding as a constraint...Figure 12.6 Overhead in terms of bandwidth introduced by security chain depl...
13 Chapter 13Figure 13.1 IoT adoption estimation for the 2017–2030 period [1].Figure 13.2 Categories of BIoT risks.Figure 13.3 BC‐IoT integration (BIoT) metrics.Figure 13.4 BC and IoT integration models [22]. (a) IoT–IoT, (b) IoT–Blockch...Figure 13.5 An SDN‐enabled fog and cloud‐based BIoT architecture.Figure 13.6 BIIT 1.0 architecture.
Guide
7 Preface
9 Acronyms
12 Index
13 WILEY END USER LICENSE AGREEMENT
Pages
1 ii
2 iv
3 xv
4 xvi
5 xvii
6 xviii
7 xix
8 xx