Data Mining and Machine Learning Applications. Группа авторов
Чтение книги онлайн.
Читать онлайн книгу Data Mining and Machine Learning Applications - Группа авторов страница 25
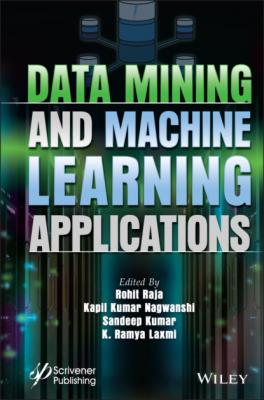
21. Wu, C.-W., Lin, Y.-F., Yu, P.S., Tseng, V.S., Mining high utility episodes in complex event sequences, in: Proceedings of the 19th ACM SIGKDD International Conference on Knowledge Discovery and Data Mining, ACM, pp. 536–544, 2013.
22. Huang, K.-Y. and Chang, C.-H., Efficient mining of frequent episodes from complex sequences. Inf. Syst., 33, 1, 96–114, 2008.
23. Beyer, K., Goldstein, J., Ramakrishan, R., Shaft, U., When Is Nearest Neighbor Meaningful? Proc. of the 7th International Conference on Database Theory, pp. 217–235, 1999.
24. Liu, H. and Yu, L., Toward Integrating Feature Selection Algorithms for Classification and Clustering. IEEE Trans. Knowl. Data Eng., 17, 3, 1–12, 2005.
25. Raja, R., Sinha, T.S., Dubey, R.P., Soft Computing and LGXP Techniques for Ear Authentication using Progressive Switching Pattern. Int. J. Eng. Future Technol., 2, 2, 66–86, 2016.
26. Raja, R., Sinha, T.S., Dubey, R.P., Orientation Calculation of human Face Using Symbolic techniques and ANFIS. Int. J. Eng. Future Technol., 7, 7, 37–50, 2016.
27. Cohena, G., Hilariob, M., Saxc, H., Hugonnetc, S., Geissbuhler, A., Learning from imbalanced data in surveillance of nosocomial infection. Intell. Data Anal. Med., 37, 1, 7–18, 2006.
28. Czyzewski, Mining Knowledge in Noisy Audio Data, in: Proc. 2nd Int. Conf. on KD and Data Mining, pp. 220–225, 1996.
29. Wang, D., Kim, Y.-S., Park, S.C., Lee, C.S., Han, Y.K., Learning-Based Neural Similarity Metrics for Multimedia Data Mining. Soft Comput., 11, 4, 335–340, February 2007.
30. Chien, S., Fisher, F., Mortensen, H., Lo, E., Greeley, R., Using Artificial Intelligence Planning to Automate Science Data Analysis for Large Image Databases, in: Proc. 3rd Int. Conf. on Knowledge Discovery and Data Mining, pp. 147–150, 1997.
31. Zaïane, O.R., Han, J., Li, Z.-N., Chee, S.H., Chiang, J.Y., MultiMediaMiner: A System Prototype for MultiMedia Data Mining, in: Intelligent Database Systems Research Laboratory and Vision and Media Laboratory report, 2009.
32. Witten, I.H., Text mining, in: Computer Science, University of Waikato, Hamilton, New Zealand, 2005.
33. Ordenoz, C. and Omiecinski, E., Discovering association rules based on image content, in: ADL ‘99: Proceedings of the IEEE Forum on Research and Technology Advances in Digital libraries, IEEE Computer Society, Washington, DC, p. 38, 1999.
34. Vijayakumar, V. and Nedunchezhian, R., A study on video data mining. Int. J. Multimed. I Inf. Retr., 1, 3, 153–172, Publisher Springer-Verlag, October 2012.
35. Brunner, R.J., Djorgovski, S.G., Prince, T.A., Szalay, A.S., Massive Datasets in Astronomy, in: Handbook of Massive Datasets, J. Abello, P. Pardalos, M. Resende, (Eds.), p. 931, Kluwer Academic Publishers, New York, 2002.
36. NASA, Science Data Users Handbook, Landsat Project Science Office. Goddard Space Flight Center, Greenbelt, 2002.
37. NASA, EOS Reference Handbook, A Guide to NASA’s Earth Science Enterprise and the Earth Observing System, EOS project science office, Greenbelt, 1999.
38. Vucetic, S., Fiez, T., Obradovic, Z., Analyzing the Influence of Data Aggregation and Sampling Density on Spatial Estimation. Water Resour. Res., 36, 12, 3721–3731, 2000.
39. Han, J., Altman, R.B., Kumar, V., Mannila, H., Pregibon, D., Emerging Scientific Applications in Data Mining. Commun. ACM, 45, 8, 54–58, 2002.
40. Brown, M.P.S., Grundy, W.N., Lin, D., Cristianini, N., Sugnet, C.W., Furey, T.S., Ares Jr., M., Haussler, D., Knowledge-based Analysis of Microarray Gene Expression Data By Using Support Vector Machines. Proc. Natl. Acad. Sci., 97, 262–267, 2000.
41. Barrett, E.C. and Curtis, F.L., Introduction to Environmental Remote Sensing, Stanley Thornes Pub. Ltd, Cheltenham, UK, 1999.
42. Kar, K. and Raja, R., A Review on Weather Prediction using Data Mining Techniques. International Conference on New Frontiers of Engineering Science, Management and Humanities (ICNFESMH-2017) Associated with national Institute of Technical Teachers Training and Research Program (NITTTR), 21 May 2019.
43. Kar, K., Raja, R., Chopra, J., Extreme Weather Event Change Prediction using CDF. International Conference on Advancement in Engineering, Applied Science and Management (ICAEASM-2017) at Centre for Development of Advanced Computing (C-DAC), Juhu, Mumbai, Maharashtra (India), pp. 448–452.
44. Nogues-Paegle, J., Mo, K., Paegle, J., Predictability of the NCEP-NCAR Reanalysis Model During Austral Summer. Mon. Weather Rev., 126, 3135–3152, 1998.
45. Kamath, C., Introduction to scientific data mining. Presented at Mathematical Challenges in Scientific Data Mining, Short Program at Institute for Pure and Applied Mathematics, Univ. of California Los Angeles, 2002.
46. Pallavi, S., Ramya laxmi, K., Ramya, N., Study and Analysis of Modified Mean Shift Method and Kalman Filter for Moving object Detection and Tracking, in: 3rd International Conference on Computational Intelligence and Informatics (ICCII-2018), held during 28–29 Dec 2018, 2018.
47. Burl, M., Kamatch, C., Kumar, V., Namburu, R., Third Workshop on Mining Scientific Datasets. First SIAM Int’l Conf. Data Mining, Chicago, IL, 2001.
48. Kumar, V., Burl, M., Kamatch, C., Namburu, R., Fifth Workshop on Mining Scientific Datasets, Second SIAM Int’l. Conf. Data Mining, Arlignton, VA, 2002.
49. Grossman, R.L., Creel, E., Harinath, S., Mazzucco, M., Reinhart, G., Turinskiy, A., Terabyte Challenge 2000: Project DataSpace. Workshop on Mining Scientific Datasets, University of Minnesota, Minneapolis, MN, 2000.
50. Kumar, S., Jain, A., Shukla, A.P., Singh, S., Rani, S., A Comparative Analysis of Machine Learning Algorithms for Detection of Organic and Non-Organic Cotton Diseases. Math. Probl. Eng., Special Issue—Deep Transfer Learning Models for Complex Multimedia Applications, vol. 1, pp. 1–18, 2021.
51. Lazarevic, A. and Obradovic, Z., Knowledge Discovery in Multiple Spatial Databases. Neural Comput. Appl., 10, 4, 339–350, 2002.
52. Roddick, J.F. and Spiliopoulou, M., A Bibliography of Temporal, Spatial, and Spatio-Temporal Data Mining Research. SIGKDD Explor., 1, 34–38, 1999.
53. Han, J., Altman, R.B., Kumar, V., Mannila, H., Pregibon, D., Emerging Scientific Applications in Data Mining. Commun. ACM, 45, 8, 54–58, 2002.
54. Niyogi, P., Girosi, F., Poggio, T., Incorporating Prior Information in Machine Learning by Creating Virtual Examples. Proc. IEEE, 86, 11, 2196–2209, 1998.
55. Domingos, P. and Hulten, G., Mining High-Speed Data Streams. Knowl. Discovery Data Min., 2, 71–80, 2000.
56. Pokrajac, D., Hoskinson, R.L., Obradovic, Z., Modeling spatiotemporal Data with a Short Observation History. Knowl. Inf. Syst., 5, pp. 368–386, 2003.
57. Hall, D. and Llinas, J., Handbook of Multisensor Data Fusion, CRC Press,