Industrial Internet of Things (IIoT). Группа авторов
Чтение книги онлайн.
Читать онлайн книгу Industrial Internet of Things (IIoT) - Группа авторов страница 12
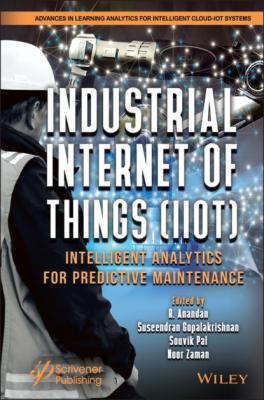
1.2.1 AI Concept
Another characteristic of the basic types of AI is purely reactive, since it acts after the perception of the problem, exemplifying an AI software that identifies the chess pieces on the board and their movement, but has no memory of past movements, ignoring everything before the current movement, that is, it only reacts to the position of the pieces on the board. In the legal field, lawyers focus on more complex aspects of law practice, given the use of text analysis, Jurimetrics, text review, data mining, contract analysis, computational argumentation, and other possible AI-derived features [17, 23], still pondering the characteristics of AI-related to its capacity for intelligent perception, such as visual perception, speech perception, auditory perception, and processing and learning of perceptual information. Reflecting on autonomous cars and virtual assistants, there is not only a programmed answer to specific questions but answers that are more personalized [23–25].
Through AI solutions, it is possible to eliminate boring tasks that may be necessary, but with machine learning, it performs basic tasks, considered human-computer interaction technologies, or even related to the more robust use found in conversational interfaces that use machine learning to understand and meet customer needs [23–25].
Even through AI solutions, it is possible to concentrate diffuse problems where data inform all levels of the operation of a modern company, i.e., it has a lot of material to interpret, so it is necessary to consume this amount of information at scale. Since the extent of the data available today has gone beyond what humans are capable of synthesizing, making it a perfect job for machine learning. Through the data, the information is extracted from various sources of public and private data, still comparing them and making changes when necessary [25].
Through AI solutions, it is possible to distribute data, given that modern cybersecurity leads to the need to compare terabytes of internal data with a quantity of external data. With machine learning, it can automate the process of detecting attacks as cybersecurity problems change and increase, vital for dealing with distributed data problems, assessing that humans are unable to involve their actions around a distribution so wide of information. AI solves dynamic data, which is a valued characteristic, given the major obstacle related to addressing individual employee characteristics, or dynamic problems of human behavior. Through AI, it is possible to use determining complex patterns to help organizations move more quickly and respond better to the changing needs of each employee [26].
Or even, through AI, industrial systems integrate robotics powered by AI, 3D printing technologies, and human supervision, building interactive robot systems leading by AI technologies. This process not only decreases costs and increases efficiency but also generates much safer industrial environments for human workers. The dangerous elements of industrial activities are surpassed by machines [27, 28].
In simpler terms, AI technologies consist of intelligent systems or intelligent machines that mimic human intelligence to operate tasks and can improve iteratively supported on the information it collects. AI technologies manifest itself in various ways in modern contemporary society as chatbots to understand customer issues more quickly and provide more efficient responses or smart assistants to analyze critical data and information from large sets of free-text data to improve programming, or even at home, through recommendation mechanisms providing intuitive recommendations for TV programs supported on users’ viewing habits. However, AI technologies are not deliberate to replace human beings but aims to substantially improve human skills and actions, tasks, and even contributions [17, 23, 24].
AI is related to application areas that involve expert systems or systems based on knowledge, natural language comprehension/translation, intelligent systems/learning, speech comprehension/generation, automatic programming, or even image and scene analysis in real time, among many others. Therefore, it can be evaluated that the technological AI field aims to emulate human beings’ capabilities including problem-solving, understanding natural language, computer vision, and robotics, considering systems for knowledge acquisition, and even knowledge representation methodologies [15].
To obtain the full value of AI, Data Science is necessary (Figure 1.3), consisting of a multidisciplinary field that employs scientific methods to collect and extract value from data, combining skills such as statistics, probabilities, frequency of occurrence of events, observational studies, and computer science, with business knowledge to analyze data gathered from distinct sources [29, 30].
The central principle of AI technologies is to replicate, and then exceed, the processes and conduct humans perceive, notice, see, and react to the world, fueled by several forms of Machine Learning techniques that recognize patterns in data to allow prognosis and predictions. Propitiate a better comprehensive understanding of the wealth of available data, information, and predictions to automate overly complex or ordinary tasks, improving productivity and performance, automating tasks or processes that previously demand human energy, and also making sense of the data on a superhuman scale [31].
Data science makes it a priority to add technological value to business intelligence and advanced analysis as the main technology differential for companies, through the use of demographic and transactional data to foresee and predict how much certain customers and users will spend over their business relationship with a company (or even the customer’s lifetime value), price optimization supported on preferences and customer behavior, or even utilizing image recognition techniques to analyze X-ray digital images searching for signs of cancer [30].
Figure 1.3 AI and data science illustration.
Three elements are leading the development of AI technologies across all sectors, which are the computational high-performance, affordable, and even processing capacity available, assessing the abundance of computing power in the cloud technologies allowing easy access to affordable and high-performance computing power. Large volumes of data available for conduct training, given that AI, require to be trained on a lot of data available to generate the correct predictions, also relating the emergence of distinct tools for labeling data, in addition to the ease and accessibility of storing and processing structured and unstructured data, to train AI algorithms [31].
The benefits of operationalizing AI are related to the cognitive interactions of machine learning techniques with conventional business applications, methods, and processes that can greatly increase productivity and user experience, or even considering AI as a strategic method and competitive advantage related to greater efficiency in processes, doing more in less time, and increasing customer loyalty, creating customized and attractive customer (user) experiences, and predicting commercial results to generate greater profitability [23, 24, 32].
AI applications in people’s daily lives are based on an app that recognizes the content of images and allows a search by typing the name of an object or action, or streaming platforms transcribing audio and generating subtitles for videos, or in an email offering automatic responses smart; or even with regard to online translators who translate texts from signs, labels, and menus with the cell phone camera; or even pondering about streaming platforms that use AI to understand users’ preferences and recommend music and movies, respectively, still relating autonomous cars that drive alone, or even in medicine, advancing cancer studies [26].
The