Multimedia Security, Volume 1. William Puech
Чтение книги онлайн.
Читать онлайн книгу Multimedia Security, Volume 1 - William Puech страница 18
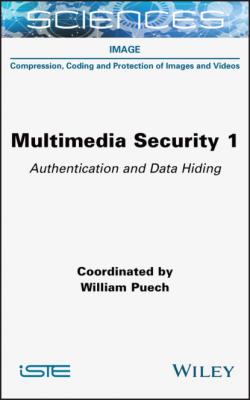
Figure 1.15. Structure of the Mayer and Stamm (2019) network to compare the source of two patches. The same first network A is applied to each patch to extract a residue. These residues are then passed on to a network B which will compare their source and decide if the patches come from the same image or not
Recently, Siamese networks have also been used for the detection of falsification (Mayer and Stamm 2019). They are bipartites, as shown in Figure 1.15. They consist of a first convolutional network that is applied independently to two image patches to extract hidden information from each, and then of a second network that compares the information extracted on the two patches to determine whether they come from the same picture. A big advantage of these methods is the ease of obtaining training data, since it is enough to have non-falsified images available and to train the network to detect whether or not the patches were obtained from the same picture. An example of detection with Siamese networks can be found in Figure 1.16.
1.8. Conclusion
In this chapter, we have described methods that analyze an image’s formation pipeline. This analysis takes advantage of alterations made by the camera from the initial raw image to its final form, usually compressed JPEG. We have reviewed the transformations undergone by the raw image, and shown that each operation leaves traces. Those traces can be used to reverse engineer the camera pipeline, reconstructing the history of the image. It can also help detect and localize inconsistencies caused by forgeries, as regions whose pipeline appears locally different than on the rest of the image. With that in mind, it is usually impossible to guarantee that an image is authentic. Indeed, a perfect falsification, which would not leave any traces, is not impossible, although it would require great expertise to directly forge a raw image – or revert the image into a raw-like state – and simulate a new processing chain after the forgery has been done. Falsifiers rarely have the patience nor the skills needed to carry out this task, however one cannot exclude that software to automatically make forged images appear authentic may emerge in the future.
Figure 1.16. Example of modification detection with the Siamese network (Mayer and Stamm 2019)
COMMENT ON FIGURE 1.16.– The forged image comes from the database associated with Huh et al. (2018). The Siamese network gives a similarity score for each patch with a reference patch. The black areas in the Siamese network result correspond to patches that are incompatible with the reference patch.
1.9. References
Agarwal, S. and Farid, H. (2017). Photo forensics from JPEG dimples. In Workshop on Information Forensics and Security. IEEE, Rennes.
Aguerrebere, C., Delon, J., Gousseau, Y., Musé, P. (2013). Study of the digital camera acquisition process and statistical modeling of the sensor raw data. Technical report [Online]. Available at: https://hal.archives-ouvertes.fr/hal-00733538.
Arias, P., Facciolo, G., Caselles, V., Sapiro, G. (2011). A variational framework for exemplar-based image inpainting. International Journal of Computer Vision, 93(3), 319–347.
Bammey, Q., Grompone von Giol, R., Morel, J.-M. (2020). An adaptive neural network for unsupervised mosaic consistency analysis in image forensics. In Conference on Computer Vision and Pattern Recognition (CVPR). IEEE.
Bianchi, T., De Rosa, A., Piva, A. (2011). Improved DCT coefficient analysis for forgery localization in JPEG images. In International Conference on Acoustics, Speech and Signal Processing. IEEE, Prague.
Bracho, R. and Sanderson, A. (1985). Segmentation of images based on intensity gradient information. Proc. IEEE Computer Society Conference on Computer Vision and Pattern Recognition. IEEE Computer Society Press, Amsterdam.
Buades, A., Coll, B., Morel, J.-M., Sbert, C. (2011). Self-similarity driven demosaicking. Image Processing On Line, 1, 51–56.
Chen, Y.-L. and Hsu, C.-T. (2008). Image tampering detection by blocking periodicity analysis in JPEG compressed images. In 10th Workshop on Multimedia Signal Processing. IEEE, Cairns.
Chen, M., Fridrich, J., Goljan, M., Lukáš, J. (2008). Determining image origin and integrity using sensor noise. IEEE Transactions on Information Forensics and Security, 3(1), 74–90.
Choi, C.-H., Choi, J.-H., Lee, H.-K. (2011). CFA pattern identification of digital cameras using intermediate value counting. In Multimedia Workshop on Multimedia and Security. ACM, New York.
Christlein, V., Riess, C., Jordan, J., Riess, C., Angelopoulou, E. (2012). An evaluation of popular copy–move forgery detection approaches. IEEE Transactions on Information Forensics and Security, 7(6), 1841–1854.
Clarke, R.J. (ed.) (1985). Transform coding of images. Astrophysics. Academic Press, London and Orlando, FL.
Colom, M. (2014). Multiscale noise estimation and removal for digital images. PhD Thesis, University of the Balearic Islands, Palma.
Colom, M. and Buades, A. (2013). Analysis and extension of the Ponomarenko et al. method, estimating a noise curve from a single image. Image Processing On Line, 3, 173–197.
Colom, M., Buades, A., Morel, J.-M. (2014). Nonparametric noise estimation method for raw images. Journal of the Optical Society of America A, 31(4), 863–871.
Cozzolino, D. and Verdoliva, L. (2020). Noiseprint: A CNN-based camera model fingerprint. IEEE Transactions on Information Forensics and Security, 15, 144–159.
Cozzolino, D., Poggi, G., Verdoliva, L. (2015a). Efficient dense-field copy–move forgery detection. IEEE Transactions on Information Forensics and Security, 10(11), 2284–2297.
Cozzolino, D., Poggi, G., Verdoliva, L. (2015b). Splicebuster: A new blind image splicing detector. In 2015 IEEE International Workshop on Information Forensics and Security. IEEE, Rome.
Desolneux, A., Moisan, L., Morel, J.-M. (2008). From Gestalt Theory to Image Analysis. Springer, New York.
Donoho, D.L. and Johnstone, I.M. (1994). Ideal spatial adaptation by wavelet shrinkage. Biometrika, 81(3), 425–455.
Donoho, D.L. and Johnstone, I.M. (1995). Adapting to unknown smoothness via wavelet shrinkage. Journal of the American Statistical Association, 90(432), 1200–1224.
Ehret, T. (2019). Robust copy-move forgery detection by false alarms control [Online]. Available at: https://arxiv.org/abs/1906.00649.
Ehret, T. and Facciolo, G. (2019). A study of two CNN demosaicking algorithms. Image Processing On Line, 9, 220–230.
Fan, Z. and de Queiroz, R.L. (2003). Identification of bitmap compression history: Jpeg detection and quantizer estimation. IEEE Transactions