The Digital Agricultural Revolution. Группа авторов
Чтение книги онлайн.
Читать онлайн книгу The Digital Agricultural Revolution - Группа авторов страница 26
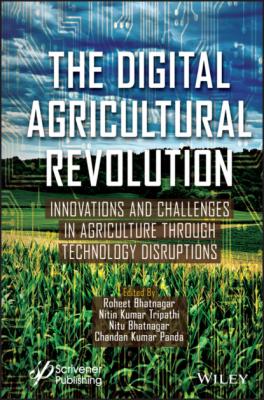
Scatter plots of predicted yield and observed yield are shown in Figures 2.10 and 2.11. This shows that the points are equally distributed over 1:1 line and also in close agreement with an R2 value of 0.9681 for paddy. There was wide scattering of yield points in case of sugarcane. There was an under estimation of yield in some cases of cane yield prediction. Although there was a deviation in crop yield prediction in few observations, the overall accuracy of the model prediction was high. The R2 value was high for paddy compared with sugarcane crop. However, a slight underestimation of yield of the sugarcane crop indicates that sensitivity of yield algorithms to crop input parameters may be further improved by changing the input parameters.
Table 2.4 Statistical analysis of neural network training and testing of season in different years.
Training | Testing | |||||||
---|---|---|---|---|---|---|---|---|
Year | RMSE | R ratio | MAE | R2 | RMSE | R ratio | MAE | R2 |
Paddy (Kharif) | 0.117 | 1.063 | 0.095 | 0.946 | 0.108 | 1.065 | 0.085 | 0.936 |
Paddy (Rabi) | 0.125 | 0.987 | 0.108 | 0.967 | 0.317 | 0.620 | 0.178 | 0.950 |
Sugarcane | 0.150 | 1.006 | 0.119 | 0.916 | 0.184 | 0.556 | 0.143 | 0.924 |
It is also observed during the study that for the model, the RMSE decreased with increased number of hidden nodes from 1 to [i + 1], where “i” is the number of input layer nodes (i = 5). Further, R2 increased and MAE decreased with the increased the number of hidden nodes from 1 to [i + 1], in all the years. The results are in accordance with researchers [74–76]. After 20 hidden nodes, the trails are conducted at a step of 10. After a number of trials with 100 to 10,000 epochs with each step of 100 up to 2000 and a step of 1000 up to 10000, better results are found at 1000 epochs for most of the cases. Although the performance increased after i+1, the computation time increased with increase in the number of nodes and epochs. The smaller the data sets, the lesser hidden nodes requirement and lower learning rates in the optimized model is observed [62]. The best performance of the models was observed at i+1 and i+2 hidden nodes. Statistical analysis revealed that the reliability of the model in crop yield estimation. The final predicted yield map of paddy and sugarcane during 2015 are shown in Figures 2.12 and 2.13. Paddy yields in the study area varied from 3.25 t/ha to 6.6 t/ha. The sugarcane yields were ranged between 70000 kg/ha and 125,000 kg/ha.
Figure 2.10 Scatter plots of actual and FFBP NN model predicted yield of sugarcane during 2015 (original figure).
Figure 2.11 Scatter plots of actual and FFBP NN model predicted yield of sugarcane during 2015 (original figure).
There was an underestimation of yield in some cases of cane yield prediction. Although there was a deviation in crop yield prediction in few observations, the overall accuracy of the model prediction was high. The model prediction accuracy may be further improved by changing the input parameters. Sugarcane crop is sensitive to leaf area index (LAI), and number of stalks per meter [77]. A stronger relationship exists between sugarcane yield and rainfall. Total soil available water is an important indicator of yield. Another important point, which differs yield prediction, is input parameter as average yield. In case of sugarcane, the input is given as average of plant and ratoon for the 3 years. The improvement of model was not attempted because of the nonavailability of the data on sugarcane crop-sensitive parameters, like the number of stalks per meter and total soil available water.