Pricing Insurance Risk. Stephen J. Mildenhall
Чтение книги онлайн.
Читать онлайн книгу Pricing Insurance Risk - Stephen J. Mildenhall страница 20
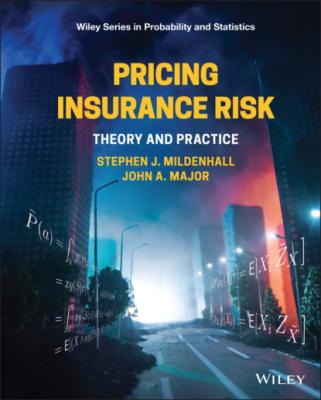
Gross | Net | |||||
---|---|---|---|---|---|---|
Statistic | Cat | Non-Cat | Total | Cat | Non-Cat | Total |
Mean | 20.000 | 80.000 | 100.000 | 17.786 | 80.000 | 97.786 |
CV | 1.000 | 0.150 | 0.233 | 0.737 | 0.150 | 0.182 |
Skewness | 3.972 | 0.300 | 2.539 | 3.139 | 0.300 | 1.351 |
Kurtosis | 35.933 | 0.135 | 19.173 | 55.220 | 0.135 | 16.336 |
Figure 2.4 shows the loss densities. The top left plot shows the different body behavior. Non-Cat is close to symmetric. Cat has a peaked distribution, with mode much lower than mean. The spike in the net plots is caused by claims generating a limit loss to the reinsurance on Cat. The different tail behavior of the two units is evident, especially on a log scale. noncat has a log concave density whereas Cat is eventually log convex. The impact of reinsurance is clear. It introduces a point mass at the aggregate attachment for Cat. The total distribution is still continuous. Figure 2.5 shows the bivariate plots. The left plot emphasizes the different tail behavior; Non-Cat remains localized close to its mean while Cat decays very slowly (vertically).
2.4.4 Hurricane and Severe Storm Case Study
In the Hu/SCS Case Study, Ins Co. has catastrophe exposures from severe convective storms (SCS) and, independently, hurricanes (Hu). In practice, hurricane exposure is modeled using a catastrophe model. We proxy that using a very severe lognormal distribution in place of the gross catastrophe model event-level output. Both units are modeled by an aggregate distribution with a Poisson frequency and lognormal severity. The key stochastic parameters are shown in Table 2.4.
The Case includes a gross and net view. Net applies per occurrence reinsurance to the Hu unit with an attachment probability 0.05 (¤ 40) and detachment probability 0.005 (¤ 413). The reinsurance assumes an unlimited number of free reinstatements, to simplify computations. In practice, the net distribution would include inuring property per risk and quota share programs. It would be taken from the net catastrophe model event-level output.
Other attritional (i.e., noncatastrophe) losses are approximately constant in comparison, therefore we can exclude them from our calculations since reasonable risk measures are translation-invariant,. Loss statistics based on an FFT approximation are shown in Table 2.7. To improve convergence the FFT approximation limits all occurrence losses to 1.2×109, which accounts for the difference in means compared to the expected 100. All graphics are based on the FFT computation, but computations are shown based on FFT and simulation approaches. Table 4.8 shows various VaR, TVaR, and EPD levels.
Table 2.7 Hu/SCS Case Study estimated mean, CV, skewness, and kurtosis by line and in total, gross, and net. Per occurrence reinsurance applied to Hu with an attachment probability 0.05 (¤ 40) and detachment probability 0.005 (¤ 413)
Gross | Net | |||||
---|---|---|---|---|---|---|
Statistic | Hu | SCS | Total | Hu | SCS | Total |
Mean | 29.727 | 69.133 | 98.860 | 18.987 | 69.133 | 88.121 |
CV | 10.923 | 0.736 | 3.324 | 16.246 | 0.736 | 3.548 |
Skewness | 121 | 24.900 | 116 | 137 | 24.900 | 132 |
Kurtosis | 27 | 9 | 26 | 32 | 9 | 31 |
Figure 2.6 shows the loss densities. These are more extreme versions of the Cat/Non-Cat Case. Cat is extremely skewed. The lognormal severity is thick-tailed for both units, though Cat is thicker. Figure 2.7 shows the bivariate plots. The right-hand plot shows the pinched behavior typical of thick-tailed distributions. A large loss combined with a small loss is the most likely nontrivial outcome. The distribution is clustered around the axes.
2.4.5 Computational Methods
All of the computations we show were completed in Python, R, or a spreadsheet—and sometimes all three. All of the simple discrete examples are easy to replicate in a spreadsheet. There is no conceptual jump from the spreadsheet examples to the R and Python Case Studies, just an increase in the numerical complexity. Once you can implement in a spreadsheet, you understand well enough to program, or instruct a programmer to create, a production implementation.
We implemented the Case Studies using Fast Fourier Transforms (FFTs) because they are fast and precise. You do not have to worry about simulation errors or wait for simulations to complete. The FFT method is described in Wang (1998a) and Mildenhall (2005). FFT methods are extremely powerful but have a fatal flaw: they assume all marginal distributions are independent. For our examples, this flaw is irrelevant. Although the Case Studies assume independent marginals for convenience, all of the methods and algorithms still apply when the marginals are not independent. Its flaw makes FFTs unsuitable for most production