Data Cleaning. Ihab F. Ilyas
Чтение книги онлайн.
Читать онлайн книгу Data Cleaning - Ihab F. Ilyas страница 8
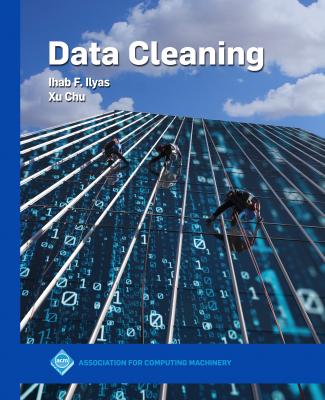
1.2.5 ML-Guided Cleaning
One can easily recognize, from the discussion so far, that ML is a natural tool to use in several tasks: classifying duplicate pairs in deduplication, estimating the most likely value for a missing value, predicting a transformation, or classifying values as normal or outliers. Indeed, several of the proposals that we discuss in this book use ML as a component in the proposed cleaning pipeline. We describe these in the corresponding chapters, and in Chapter 7 detail the use of ML for cleaning. However, with the rapid advancement in scaling inference and deploying large-scale ML models, new opportunities arise, namely, treating data cleaning as an ML task (mainly statistical inference), and dealing with all the aforementioned problems holistically. HoloClean [Rekatsinas et al. 2017a] is one of the first ML-based holistic cleaning frameworks, built on a probabilistic model of unclean databases [De Sa et al. 2019]. We discuss this direction in detail in Chapter 7.
1.2.6 Human-Involved Cleaning
It is usually impossible for machines to clean data errors with 100% confidence; therefore, human experts are often consulted in a data cleaning workflow to resolve various kinds of uncertainties whenever possible and feasible. For example, the metadata (e.g., keys and integrity constraints) generated by the discovery and profiling step need to be verified by humans before they can be used for detecting and repairing errors, since the metadata is discovered based on one instance of the data set and may not necessarily hold on any data instance of the same schema (e.g., future data). Another example of “judicious” human involvement is in the error detection step: to build a high-quality classifier to determine whether a pair of records are duplicates, we need enough training data, namely, labeled records (as duplicates or as non-duplicates). However, there are far more non-duplicate record pairs than duplicate record pairs in a data set; asking humans to label a random set of record pairs would lead to a set of unbalanced training examples. Therefore, humans need to consulted in an intelligent way to solicit training examples that are most beneficial to the classifier.
Humans are also needed in the error repair step: data updates generated by automatic data repair techniques are mostly based on heuristics and/or statistics, such as minimal repair distance or maximum likelihood estimation, and thus are not necessarily correct repairs. Therefore, they must be verified by humans before they can be applied to a data set. We highlight when and how humans need to be involved when we discuss various cleaning proposals in this book.
1.2.7 Structure of the Book
We present the details of current proposals addressing the topics discussed earlier in multiple chapters organized along two main axes, as shown in Figure 1.2: (1) task: detection vs. repair; and (2) approach: qualitative vs. quantitative. For example, outlier detection is a quantitative error detection task (Chapter 2); data deduplication is a qualitative data cleaning task (Chapter 3), which has both detection of duplicates and repair (also known as record consolidation); data transformation is considered a repair task (Chapter 4) that can be used to fix many types of errors, including outliers and duplicates; and rule-based data cleaning is a qualitative cleaning task (Chapter 6). In addition, a discovery step is usually necessary to obtain data quality rules necessary for rule-based cleaning (Chapter 5). Finally, we include a chapter that discusses the recent advances in ML and data cleaning (Chapter 7) spanning both dimensions.
Figure 1.2 Structure of the book organized along two dimensions: task and approach.
Due to the diverse challenges of data cleaning tasks, different cleaning solutions draw principles and tools from many fields, including statistics, formal logic systems such as first-order logic, distributed data processing, data mining, and ML. Although a basic familiarity with these areas should facilitate understanding of this book, we try to include necessary background and references whenever appropriate.
1. https://www.kaggle.com/surveys/2017
2. https://www.nytimes.com/2014/08/18/technology/for-big-data-scientists-hurdle-to-insights-is-janitor-work.html
3. https://www.forbes.com/sites/gilpress/2016/03/23/data-preparation-most-time-consuming-least-enjoyable-data-science-task-survey-says/
5. https://www.microsoft.com/en-us/research/project/transform-data-by-example/
2
Outlier Detection
Quantitative error detection often targets data anomalies with respect to some definition of “normal” data values. While an exact definition of an outlier depends on the application, there are some commonly used definitions, such as “an outlier is an observation which deviates so much from other observations as to arouse suspicions that it was generated by a different mechanism” [Hawkins 1980] and “an outlier observation is one that appears to deviate markedly from other members of the sample in which it occurs” [Barnett and Lewis 1994]. Different outlier detection methods will generate different “candidate” outliers, possibly along with some confidence scores.
Many applications of outlier detection exist. In the context of computer networks, different kinds of data, such as operating system calls and network traffic, are collected in large volumes. Outlier detection can help with detecting possible intrusions and malicious activities in the collected data. In the context of credit card fraud, unauthorized users often exhibit unusual spending patterns, such as a buying spree from a distant location. Fraud detection refers to the detection of criminal activities in such financial transactions. Last, in the case of medical data records, such as MRI scans, PET scans, and ECG time series, automatic identification of abnormal patterns or records in these data often signals the presence of a disease and can help with early diagnosis.
There are many challenges in detecting outliers. First, defining normal data patterns for a normative standard is challenging. For example, when data is generated from wearable devices, spikes in recorded readings might be considered normal if they are within a specific range dictated by the sensors used. On