Periodontitis and Systemic Diseases. Группа авторов
Чтение книги онлайн.
Читать онлайн книгу Periodontitis and Systemic Diseases - Группа авторов страница 4
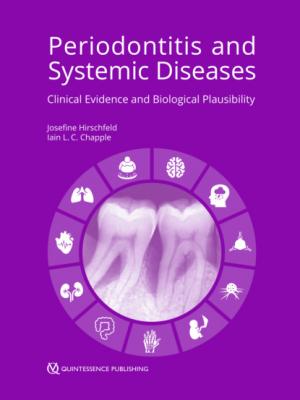
Next is the distinction between an association and a causal relationship between two or more diseases. An association is when two conditions are related such that they are commonly observed together. A causal relationship between two conditions implies that a change in one is caused by a change in the other. Causal relationships are stronger than associations, but also more difficult to prove. An example for distinguishing between these two is the following fictional research question: if researchers included coffee drinkers and non-coffee drinkers in their cross-sectional study, they may find that a greater proportion of coffee drinkers have periodontitis, compared with non-coffee drinkers. This is an association, which does not imply that coffee drinking causes periodontitis, but merely that coffee drinking and periodontitis are commonly observed together.
What would be more interesting, however, is whether coffee drinking is a component cause or part of the causal pathway of periodontitis. The causal argument can be strengthened in cross-sectional studies by accounting for things that might confound the association. In our example, it may be that people who drink coffee have higher stress levels or are more likely to smoke and therefore more likely to have periodontitis. Hence, smoking and/or stress is confounding the association observed. There are several ways of eliminating or minimising the effect of confounders. If they are known and measurable, they can be eliminated in the design of the study, for instance by excluding smokers and observing whether non-smoking coffee drinkers have a higher prevalence of periodontitis than non-smoking non-coffee drinkers.
Another method of minimising the effects of confounding is using a stratified analysis. If our fictional research was conducted in smokers as well as non-smokers, the results could be analysed separately in both groups. If the association was then found to be of a similar magnitude in both, smoking would be unlikely to be a confounder of the relationship. A further method of accounting for the potential presence of confounders is in the statistical analyses by employing regression modelling techniques, sometimes referred to as ‘adjusted analyses’. The basic premise of all these techniques, however, is that the confounder needs to be known, and needs to be easily, accurately and reproducibly measurable. This can sometimes be difficult, for example in the case of determining the socio-economic status of study participants. The ideal way of eliminating the problem of confounding is the process of randomisation. If the randomisation is robust, the arms should be equivalent in both known and unknown confounders, hence comparisons between the arms are devoid of these problems. Thus, randomised controlled trials and meta-analyses thereof form the pinnacle of the evidence-based medicine pyramid.
Fig 0-2 Bradford-Hill criteria: the nine aspects of association providing epidemiological evidence of a causal relationship between a presumed cause and an observed effect.
The criteria set out by Austin Bradford Hill, in 1965, represent a useful tool in guiding from association to causation (Fig 0-2). In this classic essay, Bradford Hill set out some aspects of association, which should be considered “before deciding that the most likely interpretation of it is causation”13. These included:
● The strength of the association. If the magnitude of the association is large, for example as measured by a high odds ratio or relative risk, it is more likely that the association will not be attenuated by some unmeasured or imperfectly measured variable. This makes the association more likely to be causal.
● Consistency. If an association has only been observed by one group at one time, it is likely to be artefactual. Reproducibility of the association from different populations at different times and or locations lends credence to the association.
● Specificity. This property relates to the association seen between two, very specific, conditions. The more specific an association between a factor and an effect, the greater the probability that it is causal. If this criterion is not met, however, it does not imply a lack of causation.
● Temporality. This criterion implies that the cause of a disease must precede the development of the disease itself. This condition is fulfilled in infection models, where exposure to a single pathogen causes a specific disease and where the exposure always precedes the disease. In complex disease processes, detecting this condition is more challenging, as the exposures are often subclinical for a period of time and may not be the sole cause, but a contributory factor.
● Biological gradient/dose–response relationship. It stands to reason that if exposure to a risk factor, pathogen or condition causes or contributes to another disease, greater exposure should be linked to poorer outcomes of that disease.
● Plausibility. Fundamental to any step from association to causation is the ability to postulate the underlying biologically plausible mechanism, by which the causal relationship is expressed. In the absence of such an explanation, implying causality becomes challenging.
● Coherence. This criterion is an extension of the plausibility criterion, stating that the plausible explanation should fit with what is currently known of the biology of the disease. Again, not meeting this criterion is not necessarily a barrier to causality, as the knowledge base is subject to evolution and change.
● Experiment. Experimentally intervening to alter the exposure to an agent suspected of contributing to a disease, and then monitoring changes in the onset or progression of that disease further strengthens the causal hypothesis.
● Analogy. If the biological mechanism from one established causal relationship is accepted, other associations employing similar biological mechanisms have a lesser burden of proof before they are accepted as causal.
The GRADE (Grading of Recommendations, Assessment, Development and Evaluations) framework is another useful tool for rating the quality of evidence in systematic reviews and other evidence syntheses, but it can also be applied also to individual studies14. It provides a systematic approach and transparent tool for generating clinical practice recommendations. Evidence from randomised controlled trials (RCTs) begins as high-quality evidence but can be downgraded according to five factors: risk of bias, inconsistency, indirectness, imprecision and publication bias. Evidence from non-randomised studies begins as low-quality evidence, but its rating can be upgraded, if no other limitations have been identified, according to three reasons: large magnitude of effect, evidence of a dose–response effect and all plausible confounding taken into account. After the process of downgrading or upgrading, the quality of the evidence for each outcome is indicated as high, moderate, low or very low15. GRADE has adopted most of Bradford Hill’s criteria, some implicitly, others explicitly. However, it has been proposed that GRADE should be adapted to consider the Bradford Hill criteria more extensively. The reason is that evidence from non-randomised studies may provide a more adequate or best available measure of a health-related research question, but that such evidence might be graded as lower quality in the GRADE framework16.
The expert