Data Lakes For Dummies. Alan R. Simon
Чтение книги онлайн.
Читать онлайн книгу Data Lakes For Dummies - Alan R. Simon страница 21
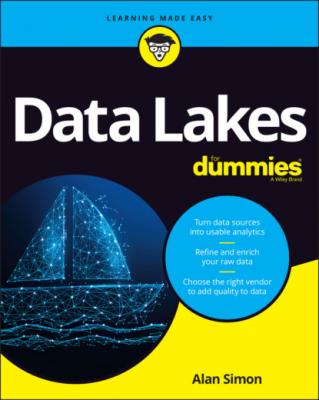
Mapping your analytics needs to your data lake road map
Jan, your CPO, is thrilled with the work that Raul and his team have done compiling the HR analytics continuum. They’ve produced an exhaustive list of more than 500 analytical functions that will be supported by the data lake, covering the broad continuum from simple “What happened?” descriptive analytics through more than a dozen complex prescriptive analytics scenarios.
Now what?
As you might guess, that 500-plus master list of HR analytics isn’t going to be available the first day your data lake goes operational. A data lake is built in a phased, incremental manner, probably over several years.
But where to start?
In Chapter 17, I show you how to build your road map that will take you from your first ideas about your data lake all the way through multiple phases of implementation.
You need the initial operating capability (IOC) of your data lake to come with some “oomph.” In other words, you want people across your organization to sit up and take notice that the data lake is, from its first days, providing some really great analytics.
You want to build your data lake using a “pipeline” approach that not only loads your data lake with lots of data but carries that data all the way through to critical business insights.
Building the best data pipelines inside your data lake
A data pipeline is an end-to-end flow of data from the original sources all the way to the end users of analytics. Figure 2-7 shows a data pipeline overlaying the journey from source systems, through the data lake’s bronze zone (the home of raw data), through the cleansing of that data into the silver zone, into the gold zone that consists of curated “packages” of data, and then finally to the users who consume the data-driven insights.
FIGURE 2-7: A data pipeline into, through, and then out of the data lake.
FIGURE 2-8: An easy way to understand data pipelines and data lakes.
Addressing future gaps and shortfalls
Your road map is only the beginning of your data lake journey. You may think you have a pretty good idea of what your data and analytical needs are over the next couple of years, and you do a good job of prioritizing the various phases of how your data lake will be built.
As your organization’s analytical needs evolve and — hopefully — become more sophisticated over time, you’ll continually adjust your data lake plans to reflect the real world.
Speedboats, Canoes, and Lake Cruises: Traversing the Variable-Speed Data Lake
You can stream all kinds of data into your data lake as quickly as that data is created in your source applications. Suppose that you dedicate a portion of your data lake to analyzing your overall computer network traffic and server performance to help you detect possible security threats, network bottlenecks, and database performance slowdowns.
You’ll be streaming tons of log data from your routers, gateways, firewalls, servers, databases — pretty much any piece of hardware in your enterprise — into your data lake, as quickly as you can as traffic flows across your network and transactions hit your databases. Then, just as quickly, you and your coworkers can analyze the rapidly incoming data and take necessary actions to keep everything running smoothly.
At the same time, not everything needs to zoom into your data lake at lightning-fast speed. Think about a lake that not only has speedboats zipping all over but also has much larger ferry-type vessels that take hundreds of passengers at a time all around the lake. Some of those ferries also offer evening gourmet dinner cruises in addition to their daytime excursions.
You’re not going to have much success trying to water-ski behind a lake ferry, nor will you have much success trying to eat a six-course gourmet meal served on the finest china while you’re bouncing all over the place on a speedboat. You need to find the proper water vessel for what you’re trying to do out on the lake, right?