The Digital Agricultural Revolution. Группа авторов
Чтение книги онлайн.
Читать онлайн книгу The Digital Agricultural Revolution - Группа авторов страница 14
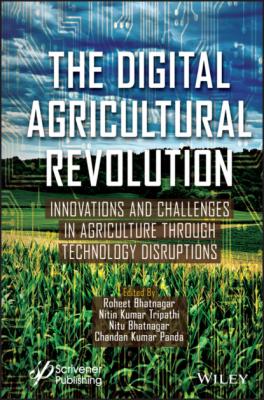
1.3.1.4 Model Development
The ultimate goal of this stage is to create, train, and test the ML model. The learning process is continued until it provides an appropriate degree of accuracy on the training data. A set of statistical processing processes is referred to as an algorithm. The type of algorithm used is determined by the kind (labeled or unlabeled) and quantity of data in the training data set, as well as the problem to be solved. Different ML algorithms are used concerning labeled data. The ML algorithm adjusts weights and biases to give accurate results.
i. Support Vector Machine
Support vector machine finds out an optimum decision boundary to divide the linear data into different classes. It is also useful to classify nonlinear data by employing the concept of kernels to transform the input data into higher dimension data. The nonlinear data will be categorized into different classes in the new higher-dimensional space by finding out an optimum decision surface.
ii. Regression Algorithm
Regression methods, such as linear and logistic regression, are used to understand data relationships. Independent variables are used to predict the value of a dependent variable using linear regression. When the dependent variable is binary, such as x or y, logistic regression can be employed. The dependency of crop yield overirrigation and fertilization is an example of linear regression. Using temperature, nitrogen, phosphorous, and potassium content in the soil, rainfall, pH of the soil as independent variables; yield can be forecasted using multiple regression.
iii. Decision Tree
The most powerful and widely used tool for classification and prediction is the DT algorithm. A DT is a tree structure that resembles a flowchart, with each leaf node representing the outcome, an inside node indicating a feature (or attribute), and a branch representing a decision rule. In a DT, the root node is the uppermost node. A Top-Down technique is used to classify the instances by sorting them down the tree from the root to a leaf node, with the leaf node provides the classification label to the given data set. This process is called recursive partitioning. Figure 1.5 shows an example of the application of the DT algorithm for the identification of leaf disease in cotton crops.
iv. K-means Clustering
It uses categorization to determine the likelihood of a data point belonging to one of two groups based on its proximity to other data points. The first stage in the k-means clustering algorithm is to determine the number of clusters (K) that will be obtained as a final result. The cluster’s centroids are then chosen at random from a set of k items or objects. Based on a distance metric, all remaining items (objects) are assigned to their nearest centroid (mostly Euclidean Distance Metric). The algorithm then calculates the new mean value of each cluster. The term “centroid update” cluster is used to build this stage. Now that the centers have been recalculated, each observation is evaluated once more to see if it is closer to a different cluster. The cluster updated means are used to reassign all of the objects. The cluster assignment and centroid update processes are done iteratively until the cluster assignments do not change anymore (until a convergence criterion is met). That is, the clusters created in the current iteration are identical to those obtained in the prior iteration. Generally, K-means clustering is used in predicting crop yields.
Figure 1.5 Cotton leaf disease using DT algorithm.
v. Association Algorithm
Association algorithms look for patterns and links in data, as well as frequently occurring “if-then” correlations known as association rules. These restrictions are comparable to data mining rules.
1.3.1.5 Improving the Model With New Data
The final stage is to apply the model to new data and, in the best-case scenario, see how accurate and effective it becomes over time. The source of the new data will be determined by the problem to be solved.
1.3.2 Artificial Neural Network
ANNs resembles the human brain based on the principle that:
Information is processed by basic units known as neurons.
Signals are transmitted from one neuron to the next via connecting links.
Each connecting link has a weight associated with it, which amplifies the signal transmitted in a conventional neural network.
To determine its output signal, each neuron’s net input passes through the activation function.
One of the popular architectures of ANN is a Multiple-layer perceptron (MLP) which consists of input, hidden, and output layers. Multiple-layer perceptrons have been successfully trained in a supervised manner utilizing a widely used method known as the Error Back Propagation Algorithm to solve a variety of complex and diverse tasks. The input layer consists of nodes that receive information from external sources and passes this information to one or more hidden layers of computation nodes and an output layer of computation nodes. During the training phase, the output is calculated for every given input and compared with the desired output. Based on the error, the network is updated. During the testing phase, the network will calculate the output for any new input data. Each conclusion has a probability assigned to it. For the most part, ANN is thought to be a good answer to difficult situations. They solve intricate relationships between crop production and interconnected characteristics that linear systems can’t solve. Artificial Neural Networks are computer programs that simulate the functioning of the human brain. Artificial Neural Network is a task-based strategy that instructs the system to work based on an internal task rather than a computationally programmed task.
1.3.2.1 ANN in Agriculture
The major advantage of neural networks is their ability to predict and anticipate via parallel thinking. Artificial Neural Network can be taught instead of being extensively programmed. Artificial Neural Network was employed by Gliever and Slaughter [30] to distinguish weeds from crops. Maier and Dandy [31] used ANNs to forecast water resources factors. Song and He [32] combined expert systems and