The Digital Agricultural Revolution. Группа авторов
Чтение книги онлайн.
Читать онлайн книгу The Digital Agricultural Revolution - Группа авторов страница 15
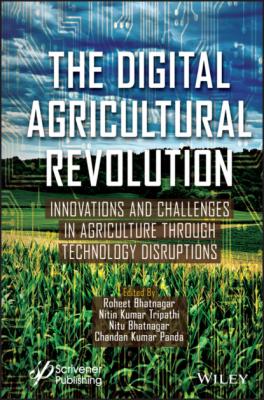
1.3.3 Deep Learning for Smart Agriculture
Images make up a significant portion of the data collected by remote sensing. Images can provide a complete view of agricultural landscapes in many circumstances, and they can help with a range of problems. As a result, imaging analysis is an important research field in the agricultural realm, and picture identification/classification is done using intelligent data analysis approaches [33]. One such approach is DL. A deep neural network is a network that has numerous hidden layers, each of which refines the preceding layer’s output. Feature learning, or the automatic extraction of features from raw data, is a key advantage of DL. This architecture finds its applications in the computer vision field for image classification, object identification, picture segmentation, and so on.
Because of the more complicated models utilized in DL, which allow huge parallelization, it can tackle more complicated problems exceptionally well and quickly [34]. Many researchers used DL for fruit counting, predicting future parameters, such as yield production, soil moisture content, evapotranspiration, weed detection, weather prediction, and so on.
1.3.3.1 Data Pre-processing
A commonly used pre-processing step is image resizing to 60 × 60, 256 × 256, 128 × 128, and 96 × 96 pixels. Image pre-processing is also used to identify the region of interest through segmentation, background removal, conversion to grayscale, and so on.
1.3.3.2 Data Augmentation
Many computer vision tasks have shown that deep neural networks perform exceptionally well. However, to avoid overfitting, huge data have to be provided to perfectly model the training data. The goal of data augmentation techniques is to artificially increase the quantity of training images. By providing the model with a variety of data, it helps to improve the overall learning method and performance, as well as generalization capability. For small data sets, this augmentation method is critical for training DL models. Some of the popular data augmentation techniques are flipping, rotating, cropping, scaling, translation, Gaussian noise, color casting, and so on.
1.3.3.3 Different DL Models
Convolutional Neural Networks (CNNs) and Recurrent Neural Networks (RNNs) are two types of DL models that are driving development in different areas including the agricultural field. Many other increasingly sophisticated architectures, such as AlexNet, VGG-16, VGG-19, ResNet, Inception-V3, DenseNet, and so on, have been developed. To apply such architectures to smaller data sets, some regularization techniques, like data augmentation, dropout, batch normalization, transfer learning, and pretraining, are implemented.
1.4 AI With Big Data and Internet of Things
Policymakers and industry leaders are turning to technology factors like Internet of Things (IoT), big data, analytics, and cloud computing to help them deal with the demands of rising food demand and climate change. Farmers can use big data to get detailed information on patterns of rainfall, fertilizer requirements, and more. This allows them to make decisions on which crops to sow for maximum profit and when to harvest. Farm yields are improved when the appropriate selections are made. Sensors have been integrated into farming equipment by companies like John Deere. This kind of monitoring can be lifesaving for big farms, as it notifies users of tractor availability, service due dates, and fuel refill warnings. In essence, this maximizes the efficiency of farm equipment while also ensuring its long-term health [38].
IoT is truly a ground-breaking modern technology due to its dynamic nature. When AI is integrated with the IoT, predictive intelligence emerges. According to some experts, IoT and AI technologies can dramatically boost crop yields and maybe the only option to reach a better system. The technology has the potential to pave the way for ecologically friendly practices as well. Figure 1.6 shows the steps involved in advanced technologies.
Farmers must keep a sharp eye on their crops for symptoms of sickness and pollution in most farming operations. The procedure is simple at a macro level, but the eyes cannot see everything. Farmers can use modern IoT solutions, as well as AI and mobile computing, to automate the entire process, leaving the review to technology. Farmers can keep track of their crops. With the help of microsensors, farmers can keep an eye on an individual plant for signs of illness or disease. Furthermore, the system can display statistics remotely via a smartphone or similar device, allowing farmers to receive real-time notifications about the condition of their fields, presence of pests, diseases, and so on.
Farmers can better safeguard their crops from pests by combining AI control technologies and IoT sensors. Farmers can use a spot treatment to treat individual plants and keep insects at bay. Simultaneously, fewer pollutants are released into the environment, particularly the soil beneath.
Figure 1.6 Steps involved in advanced technologies.
Aerial drones can evaluate and monitor crops in addition to—or perhaps instead of—IoT monitoring. The drones collect information about plants down to a single leaf using cameras and sensors placed inside. All of the acquired data, when fed into an ANN or ML solution, can produce a detailed image of a farmer’s herd.
Earth observation satellites have recently made high spatiotemporal remote sensing data available. Satellite and aerial imaging technologies are particularly valuable for capturing effective sensory images to monitor the environment, floods, fires, droughts, and other natural disasters, as well as agricultural applications like mapping, crop evaluation, crop health, and drought prediction. It offers high-speed spatial data at the global level. Numerous agricultural and hydrological indices have been created from this distant data to define the state of the land surface, primarily vegetation, groundwater level, soil moisture, and so on, to monitor and detect the beginning, duration, and severity of drought.
Managing cattle and livestock is no easy task. Farmers must not only keep track of each animal’s whereabouts, but they must also stay updated about their health. Farmers tie their cows with Fitbit-like IoT wristbands that monitor data in real-time to relieve some of the burdens. Animals will be benefitted from such wearable devices.
Experts can utilize the data acquired to develop predictive models and compare the performance to gain insights. The sharing of thousands of setups and pertinent facts in the farming world can lead to more efficient operations across the board. Agricultural specialists can exchange and consume a large quantity of knowledge, which includes anything from soil and seed tests to yield large production.
1.5 AI in the Lifecycle of the Agricultural Process
Despite modern technologies, instability in climate and unsustainable agriculture practices cause agricultural distress. The AI technology can help agriculture in the sectors [5] as shown in Figure 1.7.