Active Learning. Burr Settles
Чтение книги онлайн.
Читать онлайн книгу Active Learning - Burr Settles страница 2
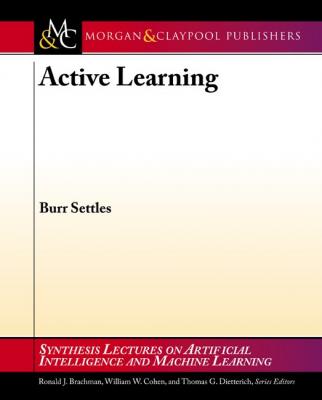
KEYWORDS
active learning, expected error reduction, hierarchical sampling, optimal experimental design, query by committee, query by disagreement, query learning, uncertainty sampling, variance reduction
Dedicated to my family and friends, who keep me asking questions.
Contents
1.3 Scenarios for Active Learning
3 Searching Through the Hypothesis Space
3.1 The Version Space
3.2 Uncertainty Sampling as Version Space Search
3.3 Query by Disagreement
3.4 Query by Committee
3.5 Discussion
4 Minimizing Expected Error and Variance
4.1 Expected Error Reduction
4.2 Variance Reduction
4.3 Batch Queries and Submodularity
4.4 Discussion
5 Exploiting Structure in Data
5.1 Density-Weighted Methods
5.2 Cluster-Based Active Learning
5.3 Active + Semi-Supervised Learning
5.4 Discussion
6.1 A Unified View
6.2 A PAC Bound for Active Learning
6.3 Discussion
7.1 Which Algorithm is Best?
7.2 Real Labeling Costs
7.3 Alternative Query Types
7.4 Skewed Label Distributions
7.5 Unreliable Oracles
7.6 Multi-Task Active Learning
7.7 Data Reuse and the Unknown Model Class
7.8 Stopping Criteria
Preface
Machine learning is the study of computer systems that improve through experience. Active learning is the study of machine learning systems that improve by asking questions. So why ask questions? (Good question.) The key hypothesis is that if the learner is allowed to choose the data from which it learns—to be active, curious, or exploratory, if you will—it can perform better with less training. Consider that in order for most supervised machine learning systems to perform well they must often be trained on many hundreds or thousands of labeled data instances. Sometimes these labels come at little or no cost, but for many real-world applications, labeling is a difficult, time-consuming, or expensive process. Fortunately in today’s data-drenched society, unlabeled data are often abundant (or at least easier to acquire). This suggests that much can be gained by using active learning systems to ask effective questions, exploring the most informative nooks and crannies of a vast data landscape (rather than randomly and expensively sampling data from the domain).
This book was written with students, researchers, and other practitioners of machine learning in mind. It will be most useful to those who are already familiar with the basics of machine learning and are looking for a thorough but gentle introduction to active learning techniques. We will assume a basic familiarity with probability and statistics, some linear algebra, and common supervised learning algorithms. An introductory text in artificial intelligence (Russell and Norvig, 2003) or machine learning (Bishop, 2006; Duda et al., 2001; Mitchell, 1997) is probably sufficient