Artificial Intelligence and Quantum Computing for Advanced Wireless Networks. Savo G. Glisic
Чтение книги онлайн.
Читать онлайн книгу Artificial Intelligence and Quantum Computing for Advanced Wireless Networks - Savo G. Glisic страница 108
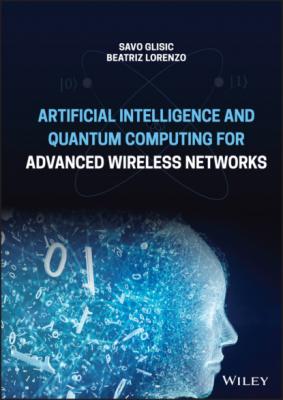
44 44 Shuman, D.I., Narang, S.K., Frossard, P. et al. (2013). The emerging field of signal processing on graphs: extending high‐dimensional data analysis to networks and other irregular domains. IEEE Signal Process. Mag. 30 (3): 83–98.
45 45 M. Defferrard, X. Bresson, and P. Vandergheynst, “Convolutional neural networks on graphs with fast localized spectral filtering,” in Proc. of NIPS, 2016, pp. 3844–3852.
46 46 M. Henaff, J. Bruna, and Y. LeCun, “Deep convolutional networks on graph‐structured data,” arXiv preprint arXiv:1506.05163, 2015.
47 47 Levie, R., Monti, F., Bresson, X., and Bronstein, M.M. (2017). Cayleynets: graph convolutional neural networks with complex rational spectral filters. IEEE Trans. Signal Process. 67 (1): 97–109.
48 48 Micheli, A. (2009). Neural network for graphs: a contextual constructive approach. IEEE Trans. Neural Netw. 20 (3): 498–511.
49 49 Y. Li, R. Yu, C. Shahabi, and Y. Liu, “Diffusion convolutional recurrent neural network: Data‐driven traffic forecasting,” in Proc. of ICLR, 2018
50 50 S. Yan, Y. Xiong, and D. Lin, “Spatial temporal graph convolutional networks for skeleton‐based action recognition,” in Proc. of AAAI, 2018.
51 51 J. Gilmer, S. S. Schoenholz, P. F. Riley, O. Vinyals, and G. E. Dahl, “Neural message passing for quantum chemistry,” in Proc. of ICML, 2017, pp. 1263–1272.
52 52 K. Xu, W. Hu, J. Leskovec, and S. Jegelka, “How powerful are graph neural networks,” in Proc. of ICLR, 2019
53 53 P. Velickovic, G. Cucurull, A. Casanova, A. Romero, P. Lio, and Y. Bengio, “Graph attention networks,” in Proc. of ICLR, 2017
54 54 S. Cao, W. Lu, and Q. Xu, “Deep neural networks for learning graph representations,” in Proc. of AAAI, 2016, pp. 1145–1152
55 55 D. Wang, P. Cui, and W. Zhu, “Structural deep network embedding,” in Proc. of KDD. ACM, 2016, pp. 1225–1234.
56 56 T. N. Kipf and M. Welling, “Variational graph auto‐encoders,” NIPS Workshop on Bayesian Deep Learning, 2016.
57 57 K. Tu, P. Cui, X. Wang, P. S. Yu, and W. Zhu, “Deep recursive network embedding with regular equivalence,” in Proc. of KDD. ACM, 2018, pp. 2357–2366.
58 58 W. Yu, C. Zheng, W. Cheng, C. C. Aggarwal, D. Song, B. Zong, H. Chen, and W. Wang, “Learning deep network representations with adversarially regularized autoencoders,” in Proc. of AAAI. ACM, 2018, pp. 2663–2671.
59 59 Y. Li, O. Vinyals, C. Dyer, R. Pascanu, and P. Battaglia, “Learning deep generative models of graphs,” in Proc. of ICML, 2018.
60 60 M. Simonovsky and N. Komodakis, “Graphvae: Towards generation of small graphs using variational autoencoders,” in ICANN. Springer, 2018, pp. 412–422
61 61 Z. Wu, S. Pan, G. Long, J. Jiang, and C. Zhang, “Graph wavenet for deep spatial‐temporal graph modeling,” in Proc. of IJCAI, 2019
62 62 Khamsi, M.A. (2001). An Introduction to Metric Spaces and Fixed Point Theory. New York: Wiley.
63 63 Powell, M.J.D. (1964). An efficient method for finding the minimum of a function of several variables without calculating derivatives. Comput. J. 7: 155–162.
64 64 Frasconi, P., Gori, M., and Sperduti, A. (1998). A general framework for adaptive processing of data structures. IEEE Trans. Neural Netw. 9 (5): 768–786.
65 65 L. Almeida, “A learning rule for asynchronous perceptrons with feedback in a combinatorial environment,” in Proc. IEEE Int. Conf. Neural Netw., M. Caudill and C. Butler, Eds., San Diego, 1987, vol. 2, pp. 609–618.
66 66 Pineda, F. (1987). Generalization of back‐propagation to recurrent neural networks. Phys. Rev. Lett. 59: 2229–2232.
67 67 Graham, A. (1982). Kronecker Products and Matrix Calculus: With Applications. New York: Wiley.
68 68 R. Singh, A. Chakraborty and B. S. Manoj, Graph Fourier Transform based on Directed Laplacian, https://arxiv.org/pdf/1601.03204.pdf
Конец ознакомительного фрагмента.
Текст предоставлен ООО «ЛитРес».
Прочитайте эту книгу целиком, купив полную легальную версию на ЛитРес.
Безопасно оплатить книгу можно банковской картой Visa, MasterCard, Maestro, со счета мобильного телефона, с платежного терминала, в салоне МТС или Связной, через PayPal, WebMoney, Яндекс.Деньги, QIWI Кошелек, бонусными картами или другим удобным Вам способом.