Artificial Intelligence and Quantum Computing for Advanced Wireless Networks. Savo G. Glisic
Чтение книги онлайн.
Читать онлайн книгу Artificial Intelligence and Quantum Computing for Advanced Wireless Networks - Savo G. Glisic страница 104
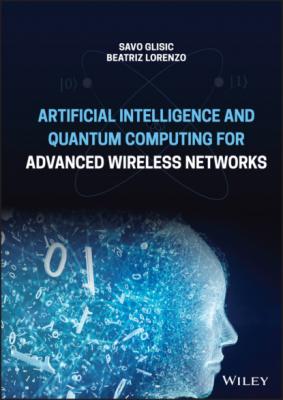
2 Instruction = z(t)(∂Fw/∂w)(x, l): By definition of Fw, fw , and BP, we have(5.92)
where y = [ln, xu, l(n, u), lu] and BP1 indicates that we are considering only the first part of the output of BP. Similarly
(5.93)
where y = [ln, xu, l(n, u), lu]. These two equations provide a direct method to compute d in positional and nonlinear GNNs, respectively.
For linear GNNs, let
holds where
where y
Time complexity of the GNN model: Formally, the complexity is easily derived from Table 5.2: it is
In positional and nonlinear GNNs, the transition function must be activated itf · ∣ N∣ and itf · ∣ E∣ times, respectively. Even if such a difference may appear significant, in practice, the complexity of the two models is similar, because the network that implements the fw is larger than the one that implements hw . In fact, fw has M(s + lE) input neurons, where M is the maximum number of neighbors for a node, whereas hw has only s +lE input neurons. A significant difference can be noticed only for graphs where the number of neighbors of nodes is highly variable, since the inputs of fw must be sufficient to accommodate the maximum number of neighbors, and many inputs may remain unused when fw is applied. On the other hand, it is observed that in the linear model the FNNs are used only once for each iteration, so that the complexity of each iteration is O(s2 ∣ E∣) instead of
In GNNs, the learning phase requires much more time than the test phase, mainly due to the repetition of the forward and backward phases for several epochs. The experiments have shown that the time spent in the forward and backward phases is not very different. Similarly, to the forward phase, the cost of the function BACKWARD is mainly due to the repetition of the instruction that computes z(t). Theorem 5.2 ensures that z(t) converges exponentially fast, and experiments have confirmed that itb is usually a small number.
Formally, the cost of each learning epoch is given by the sum of all the instructions times the iterations in Table 5.2. An inspection of the table shows that the cost of all instructions involved in the learning phase are linear with respect to both the dimension of the input graph and of the FNNs. The only exceptions are due to the computation of z(t + 1) = z(t) · A + b, (∂Fw/∂x)(x, l) and ∂pw/w, which depend quadratically on s.
The most expensive instruction is apparently the computation of ∂pw/w in nonlinear GNNs, which costs O
Appendix 5.A Notes on Graph Laplacian
Let G = (V, E) be an undirected graph with vertex set V = {v1, …, vn}. In the following, we assume that the graph G is weighted, that is, each edge between two vertices vi and vj