The Science of Reading. Группа авторов
Чтение книги онлайн.
Читать онлайн книгу The Science of Reading - Группа авторов страница 56
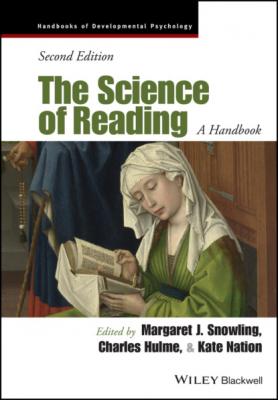
Figure 5.1 Dual pathway model of reading.
Our understanding of the nature of the spelling‐meaning mapping and its acquisition is relatively poor (Nation, 2009; Nation, 2017; Taylor et al., 2015), perhaps because it has been difficult to find a clear behavioural measure of this pathway (Seidenberg, 2011). Behavioral and electrophysiological data suggest that semantic information is activated rapidly in visual word recognition (Balota et al., 2004; Carreiras, Armstrong, Perea, & Frost, 2014), although these semantic effects may be at least in part driven by processing along the phonological pathway (van Orden, 1987). However, there is also evidence that by the age of 7, children activate semantic information from subword orthographic patterns in reading, irrespective of whether those subword patterns share pronunciation with the carrier word (e.g., the crow in crown; Nation & Cocksey, 2009). These data provide evidence for a direct mapping between spelling and meaning.
Neural models conceptualize the ventral (spelling‐to‐meaning) pathway as supporting reading expertise (Dehaene‐Lambertz, Monzalvo, & Dehaene, 2018; McCandliss, Cohen, & Dehaene, 2003). Likewise, longitudinal data suggest that sensitivity along this pathway continues to develop into adolescence (Ben‐Shachar, Dougherty, Deutch, & Wandell, 2011) as reliance shifts from the dorsal pathway to the ventral pathway (Pugh et al., 2000). More recent neuroimaging research has revealed a hierarchical posterior‐to‐anterior gradient whereby representations of visual symbols become increasingly invariant as they are transformed to meaningful information (Taylor, Davis, & Rastle, 2019).
Morphology brings an important dimension to thinking about the nature of the spelling‐meaning mapping. Recent estimates suggest that the average 20‐year‐old English speaker recognizes around 71,400 printed words (Brysbaert, Stevens, Mandera, & Keuleers, 2016). If the spelling‐meaning mappings were wholly arbitrary, this would require children to learn over 12 new printed words each day (assuming that learning to read begins at age 4); surely, an unfeasible task. However, if we remove inflectional variants of stems (e.g., cleans, cleaning, cleaned), this reduces the learning challenge to 42,000 printed words. If we go further and remove derivational variants (e.g., unclean, cleanliness, cleanly), this reduces the learning challenge to 11,100 stems (Brysbaert, et al., 2016; see also Nagy & Anderson, 1984), or to just under two new words each day. Though this figure seems more feasible, it is still over double the number of distinct spellings required for full literacy in Chinese (Katz & Frost, 1992), providing an indication of the difficulty of acquiring the spelling‐meaning mapping. Crucially, these revised figures also presume that the learner has sufficient knowledge of morphological relationships to recognize that printed words such as cleanly, unclean, cleanliness, and cleaned are variations of clean.
The previous discussion suggests that acquiring knowledge of morphological relationships may be a vital part of developing a direct mapping between spellings and meanings. Strong causal evidence for this hypothesis is lacking. However, neural evidence using MEG (Lewis, Solomyak, & Marantz, 2011; Solomyak & Marantz, 2010), fMRI (Devlin, Jamison, Matthews, & Gonnerman, 2004; Gold & Rastle, 2007), and diffusion tensor imaging (Yablonski, Rastle, Taylor, & Ben‐Shachar, 2019) converge in suggesting that morphological computations engage ventral (spelling‐meaning) pathway regions of the reading system (Rastle, 2019a).
Morphological Analysis in Skilled Reading
There is strong evidence that morphological information is analysed during the recognition of printed words. Much of this evidence derives from three paradigms: the morpheme frequency paradigm, the morpheme interference paradigm, and the morphological priming paradigm. This evidence is reviewed briefly in the following section (see also Amenta & Crepaldi, 2012 for fuller review).
The term morphological decomposition is typically used to describe the analysis of morphemic information. This term has usually been used to refer to a process of segmenting words into morphemic constituents (e.g., segmenting unclean into the constituents [un‐] + [clean]) during the recognition process (e.g., Taft, 1994; Taft & Forster, 1975). However, the term morphological decomposition can also refer to the way that morphologically complex words are represented in distributed‐connectionist networks (e.g., Rastle & Davis, 2008). In these networks, if a surface pattern occurs in many words in a semantically consistent manner (e.g., the clean in unclean, cleanly, cleaner, and cleanliness), then the learned distributed representations will come to treat that pattern independently of the rest of the word (Plaut & Gonnerman, 2000). These different characterizations of morphology are discussed later in this chapter. My use of language regarding morphological decomposition should not imply any specific theoretical perspective at this stage.
Morpheme frequency effects
Several studies have reported that visual word recognition is influenced by the frequency of the stem within a morphologically complex word (e.g., Taft, 1979). Typically, participants in these experiments are asked to make lexical decisions for sets of words matched on whole‐word frequency but varied on the frequency of stems (e.g., unreal versus refuel; Taft & Ardasinski, 2006). Results show faster lexical decision times for words with high‐frequency stems. This result has been demonstrated for both inflectional and derivational morphology, and across a number of different languages, including Italian (Burani, Salmaso, & Carramazza, 1984), Dutch (Baayen, Dijkstra, & Schreuder, 1997), and French (Colé, Beauvillain, & Segui, 1989). The fact that recognition time of morphologically complex words is based at least in part on the frequency of the stem (e.g., the real in unreal) indicates that the word recognition system is sensitive to morphological structure.
More recent research has begun to indicate that these morpheme frequency effects might be modulated by other factors. Baayen, Wurm, and Aycock (2007) used lexical decision data from the English Lexicon Project (Balota et al., 2004) to suggest that the effect of morpheme frequency might be restricted to words with low whole‐word frequency. This finding is unsurprising given that the recognition of low frequency words is slower and less accurate than for high frequency words; thus, there is less pressure in the system to represent morphemic constituents when words are high in frequency. Likewise, there is some evidence to suggest that stem frequency effects are larger when derived target words contain highly productive affixes (Bertram, Schreuder, & Baayen, 2000; Ford, Davis, & Marslen‐Wilson, 2010). Productive affixes tend to occur frequently, in combination with many different stems and in semantically consistent ways (e.g., ‐ness as in kindness is productive; ‐th as in warmth is not).
The morpheme frequency effects that I have described seem straightforward, but there is an important methodological point to consider. Typically, these experiments compare the recognition of words matched on whole‐word frequency while varying stem frequency. However, the way that we count stem frequency entails theoretical commitments. Is stem frequency simply the number of times that a particular stem occurs in a corpus of a certain size? Or, should stem frequency also include the frequency of the morphologically complex words in which it occurs? If readers analyse morphologically complex words in terms of their constituents, then the latter approach would seem to be the more valid, since each encounter with a morphologically complex word reinforces knowledge of its stem. Taking this argument further, if decomposition arises in some morphologically complex words more than others (e.g., those with highly productive affixes), then that may also influence how we should quantify stem frequency. Researchers do not always think about how their decisions to match stimuli on psycholinguistic properties reflect underlying theoretical commitments. In this instance, theoretical uncertainties make it difficult to design and interpret experiments of this nature.