The Science of Reading. Группа авторов
Чтение книги онлайн.
Читать онлайн книгу The Science of Reading - Группа авторов страница 58
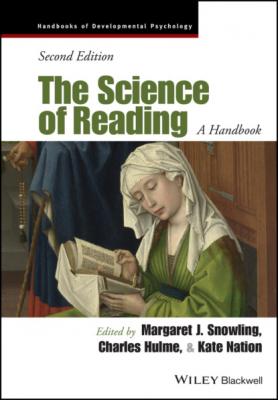
Lavric and colleagues (2012) tested this account by studying brain potentials as participants made lexical decisions to morphologically complex words (e.g., darkness), pseudo‐morphological words (e.g., corner), and nonmorphological stimuli (e.g., brothel). Results revealed that within the first 190 milliseconds, neural responses to stimuli in the two morphologically structured conditions were similar, and both differed significantly from neural responses to stimuli in the nonmorphological condition. Evidence of semantic involvement was observed 60 to 70 milliseconds later, when neural responses to stimuli in the pseudo‐morphological condition diverged from those in the other two conditions (Lavric, Elchlepp, & Rastle, 2012). The authors suggested that this second phase of recognition is suggestive of some type of process to repair the incorrect segmentation (e.g., corner is not “someone who corns”). These findings support a hierarchical model in which morphological decomposition is based initially on an orthographic analysis of morphemes, and is only later constrained by semantic information (see also Whiting, Shtyrov, & Marslen‐Wilson, 2014, for similar results using MEG methods). Conversely, these findings would appear to rule out any account in which the analysis of morphological information arises subsequent to lexical identification, such as the supra‐lexical model (Giraudo & Grainger, 2000).
Most theories of morphological processing are located within a localist tradition of modeling. These theories conceptualize morphemes as a layer of representation that resides between letter units and an orthographic lexicon (e.g., Crepaldi, Rastle, Coltheart, & Nickels, 2010; Taft, 1994; Taft & Nguyen‐Hoan, 2010). On these accounts, letter strings comprising a morphological structure such as darkness and corner would activate constituent morphemes prior to the activation of whole words, as in Figure 5.2. It is the activation of these units prior to lexical identification that is presumed to underlie the morpheme frequency effect and the morpheme interference effect. Likewise, activation of a morpheme representation due to presentation of a morphologically structured prime should facilitate later recognition of a morphologically related target. However, this type of model has never been specified computationally, and attempting to do so would immediately reveal gaps in our understanding and implementational challenges. One example of this is provided by the research testing whether sensitivity to morphemes is position specific (Crepaldi et al., 2010). This research emerged directly from an initial attempt by Davide Crepaldi and myself to specify this theory computationally within an interactive activation framework. We had not even considered the question until faced with a decision about how to implement a morpheme layer of representation in this model.
Figure 5.2 Theory of morphological decomposition based on classical localist approach.
Figure 5.3 A theory of morphological processing based on a distributed‐connectionist approach.
Alternative approaches to morphological processing are superior because they are computationally specified, but their ability to account for critical data has not been established. One perspective arises within the distributed‐connectionist modeling tradition (Harm & Seidenberg, 2004; Plaut & Gonnerman, 2000; Rueckl & Raveh, 1999; see Figure 5.3). These models reject the notion of explicit morphological representations. Instead, in learning the mapping between orthography and meaning, these models come to represent morphologically complex words componentially – that is, in terms of their morphemes (Plaut & Gonnerman, 2000). This means that the distributed representations for morphologically complex words such as darkness will overlap those of their stems (dark), providing scope for modeling morphological effects (Plaut & Gonnerman, 2000). However, these representations develop only to the extent that the morphologically complex word is related in meaning to its stem. Componential representations would not be expected to develop for pseudo‐morphological words (e.g., corner) or for a morphologically complex word with a historical relationship to its stem (e.g., witness). To date, distributed‐connectionist models do not therefore provide a ready explanation for morpho‐orthographic segmentation (Rastle et al., 2004; Rastle & Davis, 2008).
Interestingly, in order to learn morphological regularities across the spelling‐meaning mapping, distributed‐connectionist models must be provided with an input representation that allows them to recognize orthographic patterns that occur repeatedly in semantically similar words (Rastle & Davis, 2008). This is nontrivial because these orthographic patterns occur in different positions within words: for example, dislike , unlike , likely, likelihood. Thus far, this problem has been dealt with by providing distributed‐connectionist models (e.g., Plaut & Gonnerman, 2000; Rueckl & Raveh, 1999) with a representation that is pre‐segmented. Essentially, in providing these models with an input representation that is already segmented into morphemes, these modelers have assumed that morpho‐orthographic segmentation has occurred (see Rastle & Davis, 2008, for discussion). It seems possible that learned orthographic representations themselves might be structured morphologically, and Rastle and Davis (2008) described three potential mechanisms that might drive this form of orthographic learning. However, computational work is needed to evaluate these proposals.
More recently, researchers have begun to use alternative computational approaches to understand morphological processing. Naïve discriminative learning models simulate the relationship between orthographic patterns and aspects of meaning (e.g., grammatical class) and propose that these relationships may explain some morphological effects (Baayen et al., 2011). Similarly, distributional semantic models suggest that the functions of morphemes and the constraints that govern their combination with stems may be captured through large‐scale analysis of text (Marelli & Baroni, 2015). Like distributed‐connectionist models, these approaches to morphological processing eschew the notion of explicit morpheme representations, and instead ascribe morphemic effects to overlap in orthographic and meaning representations. Thus, these models may also struggle to account for morpho‐orthographic segmentation effects. However, proponents of these models emphasize that there is considerable heterogeneity amongst morphologically structured words that are not clearly related to their stems. Some of the words used in the relevant experiments (e.g., Rastle et al., 2004) have a subtle relationship to their stems; for example, an initiative that is fruitless might be described as one that didn’t bear any fruit. Likewise, while the word cryptic is unrelated in meaning to crypt, the suffix ‐ic functions in the appropriate manner grammatically (i.e., forming adjectives from nouns). However, evidence that genuinely pseudo‐morphological words such as corner are segmented (e.g., Longtin et al., 2003) would seem to pose a challenge for these models.
Mechanisms for Acquiring Morphological Knowledge
Evidence from skilled adult readers suggests that they have acquired morphological knowledge that is applied to any morphologically structured stimulus, irrespective of its lexical status. One question that arises immediately is why readers