Mind+Machine. Vollenweider Marc
Чтение книги онлайн.
Читать онлайн книгу Mind+Machine - Vollenweider Marc страница 4
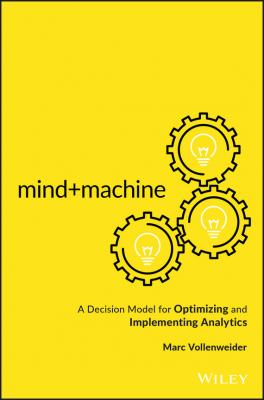
Broadly speaking, tech geeks define big data in terms of volumes; velocity (speed); variety (types include text, voice, and video); structure (which can mean structured, such as tables and charts, or unstructured, such as user comments from social media channels); variability over time; and veracity (i.e., the level of quality assurance). There are two fundamental problems with this definition. First, nobody has laid down any commonly accepted limits for what counts as big or small, obviously because this is a highly moving target, and second, there is no clear “so what?” from this definition. Why do all of these factors matter to the end user when they are all so variable?
That brings us to the anthropologist's view, which focuses on the objective. Wikipedia provides an elegant definition that expresses the ambiguity, associated activities, and ultimate objective:
Big data is a term for data sets that are so large or complex that traditional data processing applications are inadequate. Challenges include analysis, capture, data curation, search, sharing, storage, transfer, visualization, querying, updating and information privacy. The term often refers simply to the use of predictive analytics or certain other advanced methods to extract value from data, and seldom to a particular size of data set. Accuracy in big data may lead to more confident decision making, and better decisions can result in greater operational efficiency, cost reduction and reduced risk.
High-ROI use cases for big data existed before the current hype. Examples are B2C marketing analytics and advertising, risk analytics, and fraud detection. They've been proven in the market and have consistently delivered value. There are also use cases for scientific research and for national security and surveillance, where ROI is hard to measure but there is a perceived gain in knowledge and security level (although this latter gain is often debated).
We've added a collection of use cases throughout this book to help give you insight into the real-world applications of what you're learning. They all follow the same format to help you quickly find the information of greatest interest to you.
Context: A brief outline of where the use case comes from: industry, business function, and geography
Business Challenge: What the solution needed to achieve for the client(s)
Solution: An illustration of the solution or processes used to create that solution
Approach: Details on the steps involved in creating the solutions along with the mind+machine intensity diagram, illustrating the change in the balance between human effort and automation at key stages during the implementation of the solution
Analytics Challenges: The key issues to be solved along with an illustration of the relative complexity of the mind+machine aspects applied in solving the case
Benefits: The positive impact on productivity, time to market, and quality, and the new capabilities stemming from the solution
Implementation: The key achievements and the investment and/or effort required to make the solution a reality (development, implementation, and maintenance, as applicable), illustrated where possible
I wanted to include some of the more exciting projects currently under development to show the possibilities of analytics. In these cases, some of the productivity gain and investment metrics are estimates and are labeled (E).
The big data hype has its origin in three factors: the appearance of new data types or sources, such as social media; the increasing availability of connected devices, from mobile phones to machine sensors; and the evolution of ways to analyze large data sets in short periods of time. The sense of possibility led to a proliferation of use cases. We cannot say how many of these untested use cases will survive. Ultimately, the question is not what can be done, but what actually delivers value to the end user.
Gartner predicts that 60 percent of big data initiatives will fail in 2017,1 and Wikibon, an open-source research firm, maintains that the average ROI for big data projects is currently only about 55 cents on the dollar spent instead of the expected $3 to $4.2 The latter assessment wasn't made by CFOs, but came directly from practitioners, who saw a “lack of compelling need” for big data in those use cases as a reason for the low returns. However, our experience is that CFOs are increasingly asking about the viability of such analytics.
For large companies, the investment in big data infrastructure and expertise can easily run into the tens of millions of dollars. It would seem obvious that prior to any such investment, the company would want to fully investigate the need, and yet in the 2012 BRITE/NYAMA “Marketing Measurement in Transition” study, 57 percent of companies self-reported that their marketing budgets were not based on ROI analysis.3
Measuring the ROI of analytics use cases is unfortunately not as easy as it sounds. This is especially true where companies have invested in infrastructure such as central data warehouses, software licenses, and data scientist teams. Properly calculating the desired impact at the use case level requires the corresponding governance and control, which is rare at this stage. In a series of initial interviews with companies that went on to become Evalueserve clients, seven areas were found to be lacking – in some cases, almost completely:
1. Governance structure for the data and use case ownership
2. Accountability for individual use cases, portfolio management, and associated economics
3. Clear definition of analytics use cases
4. Objectives and intended end user benefits for each use case
5. Tracking the actual results against the targets
6. Knowledge management allowing the efficient reuse of prior work
7. Audit trails for the people, timing, actions, and results regarding the code, data, and findings
That said, examples of excellent and highly focused big data use case management do exist. The use case Cross-Sell Analytics: Opportunity Dashboard shows solid accountability. The campaign management function of the bank continually measures the ROI of campaigns end to end, and has built a focused factory for a portfolio of such analytics.
An example of a much weaker big data use case was recently proposed to me by a US start-up engaged in human resources (HR) analytics. The example illustrates some of the fundamental issues with the current hype. An ex-consultant and
1
“Gartner Predicts 2015: Big Data Challenges Move from Technology to the Organization,” Gartner Inc., 2014, https://www.gartner.com/doc/2928217/predicts-big-data-challenges.
2
Jeff Kelly, “Enterprises Struggling to Derive Maximum Value from Big Data,” Wikibon, 2013, http://wikibon.org/wiki/v/Enterprises_Struggling_to_Derive_Maximum_Value_from_Big_Data.
3
Columbia Business School's annual BRITE conference, BRITE–NYAMA Marketing Measurement in Transition Study, Columbia Business School, 2012, www8.gsb.columbia.edu/newsroom/newsn/1988/study-finds-marketers-struggle-with-the-big-data-and-digital-tools-of-today.