Mind+Machine. Vollenweider Marc
Чтение книги онлайн.
Читать онлайн книгу Mind+Machine - Vollenweider Marc страница 5
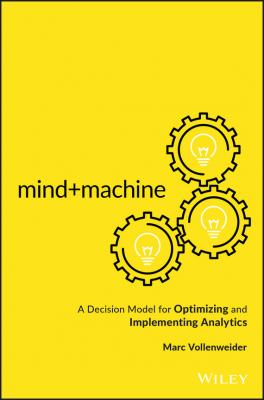
We rejected the proposal for two reasons: the obvious issue of data privacy and the expected ROI. Having done thousands of interviews, I have a very simple view of resumes. They deliver basic information that's been heavily fine-tuned by more or less competent coaching, and they essentially hide the candidate's true personality. I would argue that the predictive value of CVs has decreased over the past 20 years. Cultural bias in CV massaging is another issue. Human contact – preferably eye contact – is still the only way to cut through these walls of disguise.
The black-box algorithm would therefore have a very severe information shortage, making it not just inefficient, but actually in danger of producing a negative ROI in the form of many wrong decisions. When challenged on this, the start-up's salesperson stated that a “human filter” would have to be applied to find the false positives. Since a black-box algorithm is involved, there is no way of knowing how the software's conclusion was reached, so the analysis would need to be redone 100 percent, reducing the ROI still further.
It was also interesting to see that this use case was being sold as big data. It's a classic example of riding the wave of popularity of a term. Even under the most aggressive scenarios, our human resources performance data is not more than 300 to 400 megabytes, which hardly constitutes big data. Always be wary of excessive marketing language and the corresponding promises!
These are just two isolated use cases, which is certainly not enough to convince anyone trained in statistics, including myself. Therefore, it is necessary to look at how relevant big data analytics is in the overall demographics of analytics. To the best of my knowledge, this is not something that has ever been attempted in a study.
At first, it's necessary to count the number of analytics use cases and put them into various buckets to create a demographic map of analytics (Figure I.2). One cautionary note: counting analytics use cases is tricky due to the variability of possible definitions, so there is a margin of error to the map, although I believe that the order of magnitude is not too far off.
Figure I.2 Demographics of Use Cases
This map illustrates my first key point: big data is a relatively small part of the analytics world. Let's take a look at the main results of this assessment of the number of use cases.
1. Globally, there are a staggering estimated one billion implementations of primary use cases, of which about 85 percent are in B2B and about 15 percent in B2C companies. A primary use case is defined as a generic business issue that needs to be analyzed by a business function (e.g., marketing, R&D) of a company in a given industry and geography. An example could be the monthly analysis of the sales force performance for a specific oncology brand in the pharmaceutical industry in Germany. Similar analyses are performed in pretty much every pharmaceutical company selling oncology drugs in Germany.
2. Around 30 percent of companies require high analytics intensity and account for about 90 percent of the primary analytics use cases. International companies with multiple country organizations and global functions and domestic companies with higher complexity are the main players here.
3. The numbers increase to a staggering 50 to 60 billion use cases globally when looking at secondary implementations, which are defined as micro-variations of primary use cases throughout the business year. For example, slightly different materials or sensor packages in different packaging machines might require variant analyses, but the underlying use case of “preventive maintenance for packaging machines” would still remain the same. While not a precise science, this primary versus secondary distinction will be very relevant for counting the number of analytics use cases in the domain of Internet of Things and Industry 4.0. A simple change in sensor configurations might lead to large numbers of completely new secondary use cases. This in turn would cause a lot of additional analytics work, especially if not properly managed for reuse.
4. Only an estimated 5 to 6 percent of all primary use cases really require big data and the corresponding methodologies and technologies. This finding is completely contrary to the image of big data in the media and public perception. While the number of big data use cases is growing, it can be argued that the same holds true for small data use cases.
The conclusion is that data analytics is mainly a logistical challenge rather than just an analytical one. Managing the growing portfolios of use cases in sustainable and profitable ways is the true challenge and will remain so. In meetings, many executives tell us that they are not leveraging the small data sets their companies already have. We've seen that 94 percent of use cases are really about small data. But do they provide lower ROI because they are based on small data sets? The answer is no – and again, is totally contrary to the image portrayed in the media and the sales pitches of big data vendors.
Let me make a bold statement that is inevitably greeted by some chuckles during client meetings: “Small data is beautiful, too.” In fact, I would argue that the average ROI of a small data use case is much higher due to the significantly lower investment. To illustrate my point, I'd like to present Subscription Management: “The 800 Bits Use Case,” which I absolutely love as it is such an extreme illustration of the point I'm making.
Using just 800 bits of HR information, an investment bank saved USD 1 million every year, generating an ROI of several thousand percent. How? Banking analysts use a lot of expensive data from databases paid through individual seat licenses. After bonus time in January, the musical chairs game starts and many analyst teams join competitor institutions, at which point the seat license should be canceled. In this case, this process step simply did not happen, as nobody thought about sending the corresponding instructions to the database companies in time. Therefore, the bank kept unnecessarily paying about USD 1 million annually. Why 800 bits? Clearly, whether someone is employed (“1”) or not (“0”) is a binary piece of information called a “bit.” With 800 analysts, the bank had 800 bits of HR information. The analytics rule was almost embarrassingly simple: “If no longer employed, send email to terminate the seat license.” All that needed to happen was a simple search for changes in employment status in the employment information from HR.
The amazing thing about this use case is it just required some solid thinking, linking a bit of employment information with the database licenses. Granted, not every use case is as profitable as this one, but years of experience suggest that good thinking combined with