Biomedical Data Mining for Information Retrieval. Группа авторов
Чтение книги онлайн.
Читать онлайн книгу Biomedical Data Mining for Information Retrieval - Группа авторов страница 11
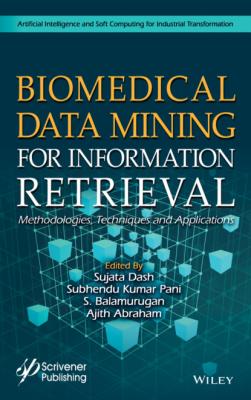
1Dept. of CSIT, Guru Ghasidas Vishwavidyalaya, Central University, Bilaspur, India
2School of Management, National Institute of Technology Karnataka, Surathkal, India
Abstract
The intensive care unit (ICU) admits highly ill patients to facilitate them serious attention and treatment using ventilators and other sophisticated medical equipments. These equipments are very costly hence its optimized uses are necessary. ICUs have a number of staffs in comparison to the number of patients admitted for regular monitoring of the patients. In brief, ICUs involve large amount of budget in comparison to other sections of any hospital. Therefore to help the doctors to find out which patient is more at risk mortality prediction is an important area of research. In data mining mortality prediction is a binary classification problem i.e. die or survive. As a result it attracts the machine learning group to apply the algorithms to do the mortality prediction. In this chapter six different machine learning methods such as Functional Link Artificial Neural Network (FLANN), Support Vector Machine (SVM), Discriminate Analysis (DA), Decision Tree (DT), Naïve Bayesian Network and K-Nearest Neighbors (KNN) are used to develop model for mortality prediction collecting data from Physionet Challenge 2012 and did the performance analysis of them. There are three separate data set each with 4000 records in Physionet Challenge 2012. This chapter uses dataset A containing 4000 records of different patients. The simulation study reveals that the decision tree based model outperforms the rest five models with an accuracy of 97.95% during testing. It is followed by the FA-FLANN model in the second rank with an accuracy of 87.60%.
Keywords: Mortality prediction, ICU patients, physioNet 2012 data, machine learning techniques
1.1 Introduction
Healthcare is the support or improvement of wellbeing by means of the avoidance, finding, treatment, recuperation or fix of sickness, disease, damage and other physical and mental hindrances in individuals [1]. Hospitals are dependent upon various weights, including restricted assets and human services assets which include limited funds and healthcare resources. Mortality prediction for ICU patients is basic commonly as the snappier and increasingly precise the choices taken by intensivists, the more the advantage for the two, patients and medicinal services assets. An emergency unit is for patients with the most genuine sicknesses or wounds. The vast majority of the patients need support from gear like the clinical ventilator to keep up typical body capacities and should be continually and firmly checked. For quite a long time, the number of ICUs has encountered an overall increment [2]. During the ICU remain, diverse physiological parameters are estimated and examined every day. Those parameters are utilized in scoring frameworks to measure the seriousness of the patients. ICUs are answerable for an expanding level of the human services spending plan, and consequently are a significant objective in the exertion to constrain social insurance costs [3]. Consequently, there is an expanding need, given the asset accessibility restrictions, to ensure that extra concentrated consideration assets are distributed to the individuals who are probably going to profit most from them. Basic choices incorporate hindering life-bolster medications and giving doesn’t revive orders when serious consideration is viewed as worthless. In this setting, mortality evaluation is an essential assignment, being utilized to foresee the last clinical result as well as to assess ICU viability, and assign assets.
In the course of recent decades, a few seriousness scoring frameworks and machine learning mortality prediction models have been developed [4]. Different traditional scoring techniques such as Acute Physiology and Chronic Health Evaluation (APACHE) [4], Simplified Acute Physiology Score (SAPS) [4], Sequential Organ Failure Assessment (SOFA) [4] and Mortality Probability Model (MPM) [4] and data mining techniques like Artificial Neural Network (ANN) [5], Support Vector Machine (SVM) [5], Decision Tree (DT) [5], Logistic Regression (LR) [5] have been used in the previous researches. Mortality prediction is still an open challenge in an Intensive Care Unit.
The objective of this chapter is to develop a model to predict whether a patient will survive in hospital or not in an ICU using different models such as Discriminate Analysis (DA), Decision Tree (DT), K-Nearest Neighbor (KNN), Naive Bayesian, Support Vector Machine (SVM) and Functional Link Artificial Neural Network (FLANN), a low complexity neural network and its comparison. The dataset have been collected from the PhysioNet Challenge 2012 [6] which consists of 4,000 records of patients admitted in ICU. There are 41 variables during first 48 h after the admission of patients to the ICU from which 5 variables indicate general descriptors—age, gender, height, ICU type and initial weight, 36 variables (time series) from which 15 variables (Temp, HR, Urine, pH, RespRate, GCS, FiO2, PaCO2, MAP, SysABP, DiasABP, NIMAP, NiDiasABP, MechVent, NISysABP) will be taken as input and 5 outcome descriptors—SAPS-1 score, SOFA score, length of stay in days (LOS), length of survival and in-hospital death (0 for survival and 1 for death in hospital) to predict the survival of patients.
The rest of the chapter is organized as follows: Section 1.2 describes the previous studies of mortality prediction, Material and methods are presented in Section 1.3 where data collection, data-preprocessing, model description is properly described. Section 1.4 presents the obtained results. Section 1.5 briefly discusses the work with conclusion and finally Section 1.6 gives the future work.
1.2 Review of Literature
Many researchers applied different models in PhysioNet Challenge 2012 dataset and obtained different accuracy results.
Silva et al. [7] have developed a method for the prediction of mortality in an in-hospital death (0 takes as survivor and 1 taken as died in hospital). They have collected the data from PhysioNet website and perform the challenges. Dataset consists of three sets: sets A, B and C. Each set has 4,000 records. The challenges are given in two events: event I for a binary classifier measurement performance and event II for a risk estimator measurement performance. For event I scoring criteria are evaluated by using sensitivity and positive predictive value and for event II Hosmer–Lemeshow statistic [8] is used. A baseline algorithm (SAPS-I) is used and obtained score of 0.3125 and 68.58 for events I and II respectively and final score they obtained for events I and II are 0.5353 and 17.58. In Ref. [9] Johnson et al. have described a novel Bayesian ensemble algorithm for mortality prediction. Artifacts and erroneous recordings are removed using data pre-processing. The model is trained using 4,000 records from training set for set A and also with two datasets B and C. Jack-knifing method is performed to estimate the performance of the model. The model has obtained values of 0.5310 and 0.5353 as score 1 on the hidden datasets. Hosmer– Lemeshow statistic has given 26.44 and 29.86 as score 2. The model has re-developed and obtained 0.5374 and 18.20 for scores 1 and 2 on dataset C. The overall performance of the proposed model gives better performance than traditional SAPS model which have some advantages such as missing data handling etc. An improved version of model to estimate the in hospital mortality in the ICU using 37 time series variables is presented in Ref. [10]. They have estimated the performance of various models by using 10-fold cross validation. In the clinical data, it is common to have missing values. These missing values are imputed by using the mean value for patient’s age and gender. A logistic regression model is used and trained using the dataset. The performance of model is evaluated by the two events: Event 1 for the accuracy using low sensitivity and positive predictive value and Event 2 for the Hosmer–Lemeshow H static model for calibration. Their model has resulted 0.516 and 14.4 scores for events 1 and 2 for test set B and 0.482 and 51.7 scores for both the event