Biomedical Data Mining for Information Retrieval. Группа авторов
Чтение книги онлайн.
Читать онлайн книгу Biomedical Data Mining for Information Retrieval - Группа авторов страница 13
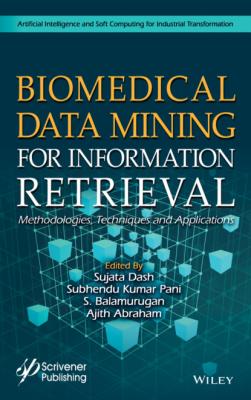
Deep learning is successfully applied in various large and complex data-sets. It is one of the new technique which is outperformed the traditional techniques. A multi-scale deep convolution neural network (ConvNets) model for mortality prediction is proposed in Ref. [29]. The dataset is taken from MIMIC-III database and 22 different variables are extracted for measurements from first 48 h for each patient. ConvNet is a multilayer neural network and discrete convolution operation is applied in the network. Convolution Neural Network models have been developed as a backend using different python packages i.e. Keras and TensorFlow. The result obtained by the proposed model gives better result of ROC AUC (0.8735, ± 0.0025) which satisfies the state of art of deep learning models.
1.3 Materials and Methods
1.3.1 Dataset
The dataset is collected from PhysioNet Challenge 2012 which consists of three sets A, B and C [6]. A total of 12,000 patient records are available. Each set consists of 4,000 records of patients from which only set A dataset of 4,000 records are used in this chapter for simulation. There are 41 variables recorded in dataset, five of these variables (age, gender, height, ICU type and initial weight) are general descriptors and 36 variables are times series variables as described in Table 1.1.
From the above 36 variables, only 15 variables are selected for mortality prediction. These variables are represented below in Table 1.2.
From these 15 variables, first value, last value, highest value, lowest value and median value are calculated for nine variables and taken as features. Only first and last values are taken for four variables. For the dataset A, five outcome-related descriptors (SAPS Score, SOFA Score, length of stay, length of survival and in-hospital death) are available from which inhospital death (0 is represented as a survivor and 1 is represented as died in hospital) is taken as a target value.
1.3.2 Data Pre-Processing
Data pre-processing is one of the technique to filter and remove noisy data. 41 variables are given in the dataset. Among them 15 variables are selected out of which some of the variables are not carefully collected and having missing values. In this chapter, missing data are replaced by zeros.
1.3.3 Normalization
All the variables in the dataset are in different ranges and in different scales. The current values of data cannot be used for classification. If all the variables have the values in better ranges and scales, classifiers will work in a better way. A standard approach, z-score normalization method is used to normalize the variables.
Table 1.1 Time series variables with description and physical units recorded in the ICU [6].
S. no. | Variables | Description | Physical units |
---|---|---|---|
1. | Albumin | Albumin | g/dL |
2. | ALP | Alkaline Phosphate | IU/L |
3. | ALT | Alanine transaminase | IU/L |
4. | AST | Aspartate transaminase | IU/L |
5. | Bilirubin | Bilirubin | mg/dL |
6. | BUN | Blood urea nitrogen | mg/dL |
7. | Cholesterol | Cholesterol | mg/dL |
8. | Creatinine | Creatinine | mg/dL |
9. | DiasABP | Invasive diastolic arterial blood pressure | mmHg |
10. | FiO2 | Fractional inspired oxygen | [0–1] |
11. | GCS | Glasgow Coma Score | [3–15] |
12. | Glucose | Serum Glucose | mg/dL |
13. | HCO3 | Serum Bicarbonate | mmol/L |
14. | HCT | Hematocrit | % |
15. | HR | Heart Rate | bpm |
16. | K | Serum Potassium | mEq/L |
17. | Lactate | Lactate | mmol/L |
18. | Mg | Serum Magnesium | mmol/L |
19. | MAP | Invasive mean arterial blood pressure | mmHg |
20. | MechVent | Mechanical Respiration Ventilation | 0/1(true/false) |
21.
|