Biomedical Data Mining for Information Retrieval. Группа авторов
Чтение книги онлайн.
Читать онлайн книгу Biomedical Data Mining for Information Retrieval - Группа авторов страница 20
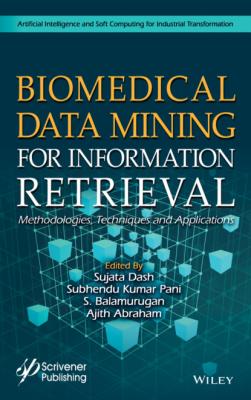
2.5 Role of Computation in Protein Structure Prediction
There are various critical and important processes and materials like personalized medicine, gene pathway, determination organs functioning, gene therapy, vaccine and drug development etc. Nowadays bioinformatics has been extensively used for the development of artificial intelligence. It also comprises softwares & programming for prediction of structure of protein however, it is still difficult to find the structure of a protein.
The two most powerful approaches are being used for determining protein structure .These are Nuclear Magnetic Resonance and X-ray crystallography but these are too expensive & time consuming which are disadvantages associated with these techniques.
Recent advancement for getting precise & fine protein structure a powerful technique has been introduced named cryo-electron microscope (Cryo-EM). This revolutionary technique predicts high resolution large scale molecular structures. The principle of this approach is mainly used in machine learning & artificial intelligence. For interpretation of cryo-EM maps, machine learning & artificial intelligence are extensively used [26–29].
Many liquid proteins cannot be crystallized. Getting Cryo-EM map crystallization of protein is mandatory. The solution of this problem can be done by AI which gives remedy for sequencing of protein without its crystallization.
Artificial intelligence has numerous programmes which are trained enough to give enormous information on atomic features of protein like: bond angles, bond length, type of bonds, physical-chemical properties, bond energy, amino acids interaction, potential energy etc. Artificial intelligence is used for image recognition [30, 31]. It helps in giving precise, broad and accurate thousands of protein structure [32, 33].
In this way these programmes suggest prediction model outputs which can be compared to the known crystal structures. There are several events organized for prediction model for protein.
Critical Assessment of Structure Prediction (CASP) is an annual gathering for comparison of protein structures by various models to assess the quality of the model and find the most accurate model making it the important milestone for protein structure prediction for multiple applications.
MULTICOM: in every two years all over the world researchers submit predicted protein structure while deep learning (Machine Learning) has been applied to make protein structure prediction with help of protein contact distance prediction. Professionals analyze the performance of these methods [34] and decide on the best models.
2.6 Application in Protein Folding Prediction
Understanding protein folding is inherent to understanding its function and its heterogenous nature. Cellular function is incomplete without proteins be it replication, transcription and translation, thus prediction of 3D or folded protein structure becomes very important to address various questions of molecular biology. Earlier various molecular biology techniques were used for determination of protein folding which was time consuming. The discovery of new protein sequences has been accelerated by next-generation sequencing techniques due to these methods being rapid and economical. The computational prediction methods that can accurately classify unknown protein sequences into specific fold categories in the shortest time possible is today’s requirement. Therefore computational recognition of protein folds holds a lot of importance in bioinformatics and computational biology. A number of efforts have led to generation of a variety of computational prediction methods and Artificial intelligence (AI) and machine learning (ML) have shown to hold great promise. In this chapter, available AI and ML methods and features have been explored and novel methods based on reinforcement learning have been discussed. Prediction of protein structure happens at four levels that is
1 i) 1-D prediction of structural features which is the primary sequence of amino acids linked by peptide bond
2 ii) 2-D prediction of which is the spatial relationships between amino acids that is alpha helix, beta turn and beta turn facilitated by hydrogen bonds
3 iii) 3-D prediction of the tertiary structure of a protein that is fibrous or globular involving multiple bonds facilitated by hydrogen bonds, Van der Wal forces, hydrophobic interactions
4 iv) 4-D prediction of the quaternary structure of a multiprotein complex which is made up of more than one peptide chain involving formation of sulfur bridge.
Thus a model development which allows the flexibility of bond formation and helps to predict a stable and functional protein structure has been facilitated to a great deal by AI and ML.
Prediction of protein structure is a complex problem as it is associated with various levels of organization and is a multi-fold process. There is a need for smart computational techniques for such purpose. AI is a great tool which when used with computational biology facilitates such prediction. Apart from determining the structure AI also aids in predicting protein structure crucial for drug development as well as in understanding the biochemical effect and ultimately the function.
A protein can be broadly described as a polymer where the individual amino acid can be considered as the monomers or the building blocks arranged in a linear chain and joined together by peptide bonds. The primary structure as described earlier is represented by a sequence of letters which represent the amino acids. The chain of amino acids of a protein folds into local secondary structures including alpha helices, beta strands, and nonregular coils [35, 36] in its native environment. The secondary structure elements are further packed to form a tertiary structure depending on hydrophobic forces and side chain interactions, such as hydrogen bonding, between amino acids [37–39]. The tertiary structure is described by the x, y and z coordinates of all the atoms of a protein or, in a coarser description, by the coordinates of the backbone atoms (Figure 2.1). The quaternary structure is formed by more than one protein chains interacting or assembling together to form a complexes structure. Theses protein complexes proteins interact with each other and with other biological macromolecules such as DNA, RNA and certain metabolites in a cell. This kind of interaction is required to carry out various types of biological functions such as enzymatic catalysis (protein complex can interact with a metal or non-metal referred to as co-enzyme), to gene regulation (interaction of transcription factors with DNA sequences), control of growth and differentiation (protein– protein interaction where ligand binding to receptor triggers a signal cascade pathway) and transmission of nerve impulses [40]. A protein’s function is and its structure are dependent on each other [37, 38, 41, 42] therefore, determination or prediction of protein structure accurately holds the key for its function determination. The most effective methods for finding protein structure since the inception of this field have been Nuclear Magnetic Resonance and X-ray crystallography which have the disadvantage of being time consuming and expensive. The recent advancement has been the introduction of cryo-electron